Image classifier-oriented metamorphic test method and system
A technology of image classifier and metamorphosis test, which is applied in software testing/debugging, instrument, character and pattern recognition, etc.
- Summary
- Abstract
- Description
- Claims
- Application Information
AI Technical Summary
Benefits of technology
Problems solved by technology
Method used
Image
Examples
Embodiment approach
[0046] Embodiment: the metamorphic relationship test determination method of image classifier (the method flow process of the embodiment of the present invention is as follows figure 1 shown), including:
[0047] 1) Combining the collected original training set S and the original test set T with the metamorphic relationship, the derived training set S' and the derived test set T' can be obtained;
[0048] 2) Secondly, the original training set S and the derivative training set S′ are respectively trained on the already written machine learning image classification algorithm A, and the image classifier AT (namely the first image classifier) and the image classifier AT′ ( i.e. the second image classifier);
[0049] 3) Test the original test set T and the derived test set T' on the image classifier AT and the image classifier AT' respectively;
[0050] 4) Judging the test results of the two classifiers O AT with O AT 'Whether it satisfies the metamorphosis relationship, so ...
Embodiment 1
[0054] Embodiment one: the metamorphosis relationship test determination method of image classifier, present embodiment is the metamorphosis test facing SVM image classifier, comprises the following steps:
[0055] First, the SVM algorithm is selected, and the data set uses the digits handwritten data set, which divides handwritten digital images into categories from 0 to 9. The machine learning algorithm is implemented based on the official Scikit-learn, a popular machine learning library. The algorithm can be divided into realization based on linear kernel function or nonlinear kernel function.
[0056] Then the metamorphosis relation is constructed for both the linear kernel function SVM algorithm and the nonlinear kernel function SVM algorithm. For training, the SVM algorithm takes labeled data of handwritten digits and learns how intrinsic properties in the training data separate the categories to derive decision boundaries. Each marker data is an image of 8 pixels by 8...
Embodiment 2
[0080] Embodiment two: the metamorphosis relation test determination method of image classifier, the present embodiment is the metamorphic test method for VGG16 image classifier, comprises the following steps:
[0081] The structure of the transformation relationship is as follows:
[0082] The second image classifier chosen is a deep learning image classifier called VGG16. The emergence of the VGG16 model has made breakthrough achievements in convolutional neural networks. The model can complete the task of image classification very well, and achieved excellent results in the 2014 ILSVRC competition. This embodiment implements VGG16 based on the tensorflow framework. The VGG16 image classifier is trained and tested on the labeled data, and the CIFAR-10 data set is selected. Each data instance of this dataset is a 32*32 pixel color image, that is, each instance has 32*32*3 feature numbers and has been divided into 10 mutually exclusive classes. Figure 6 A visualization of...
PUM
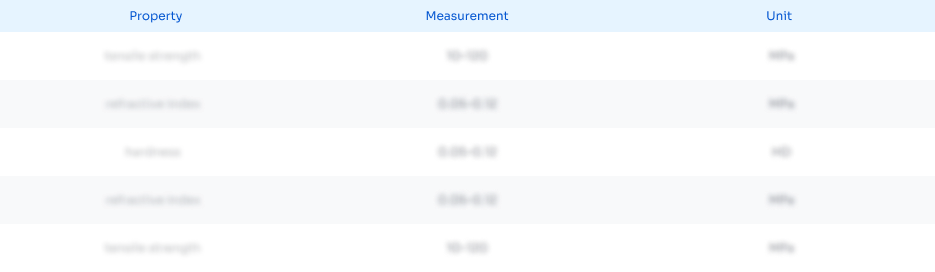
Abstract
Description
Claims
Application Information
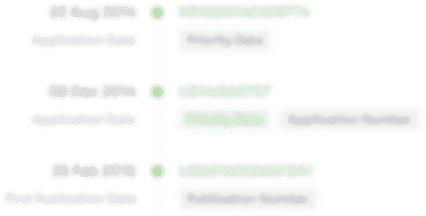
- R&D Engineer
- R&D Manager
- IP Professional
- Industry Leading Data Capabilities
- Powerful AI technology
- Patent DNA Extraction
Browse by: Latest US Patents, China's latest patents, Technical Efficacy Thesaurus, Application Domain, Technology Topic, Popular Technical Reports.
© 2024 PatSnap. All rights reserved.Legal|Privacy policy|Modern Slavery Act Transparency Statement|Sitemap|About US| Contact US: help@patsnap.com