EfficientNet-based nut surface defect classification method
A technology of defect classification and nuts, which is applied in image analysis, image data processing, instruments, etc., can solve problems such as low accuracy, low efficiency, and poor generalization ability, and achieve the effect of avoiding time-consuming, avoiding impact, and enhancing adaptability
- Summary
- Abstract
- Description
- Claims
- Application Information
AI Technical Summary
Problems solved by technology
Method used
Image
Examples
Embodiment 1
[0033] A kind of nut surface defect classification method based on EfficientNet, comprises the following steps:
[0034] Step 1: Collect training samples and make a data set; train the EfficientNet model through the data set, and classify the nut surface after the model training converges;
[0035] Step 2: collect the target image, and crop the target image;
[0036] Step 3: Modify the loss function to focal loss, adjust the positive and negative sample weights, and improve the model accuracy;
[0037] Step 4: Image preprocessing, making a template image, performing rotation correction on the input image, and making a difference to obtain a difference image, and merging the three into a three-channel image as the model input image;
[0038] Step 5: Model inference, use the EfficientNet model to infer the input image;
[0039] Step 6: Output the inference image in step 5 to the host computer for display.
[0040] Specifically, in step 1, training samples are collected, and a...
Embodiment 2
[0043] A kind of nut surface defect classification method based on EfficientNet, comprises the following steps:
[0044] Step 1: Collect training samples and make a data set; train the EfficientNet model through the data set, and classify the nut surface after the model training converges;
[0045] Step 2: collect the target image, and crop the target image;
[0046] Step 3: Modify the loss function to focal loss, adjust the positive and negative sample weights, and improve the model accuracy;
[0047] Step 4: Image preprocessing, making a template image, performing rotation correction on the input image, and making a difference to obtain a difference image, and merging the three into a three-channel image as the model input image;
[0048] Step 5: Model inference, use the EfficientNet model to infer the input image;
[0049] Step 6: Output the inference image in step 5 to the host computer for display.
[0050] Specifically, in step 2, adjust the size of the image by using...
Embodiment 3
[0052] A kind of nut surface defect classification method based on EfficientNet, comprises the following steps:
[0053] Step 1: Collect training samples and make a data set; train the EfficientNet model through the data set, and classify the nut surface after the model training converges;
[0054]Step 2: collect the target image, and crop the target image;
[0055] Step 3: Modify the loss function to focal loss, adjust the positive and negative sample weights, and improve the model accuracy;
[0056] Step 4: Image preprocessing, making a template image, performing rotation correction on the input image, and making a difference to obtain a difference image, and merging the three into a three-channel image as the model input image;
[0057] Step 5: Model inference, use the EfficientNet model to infer the input image;
[0058] Step 6: Output the inference image in step 5 to the host computer for display.
[0059] Specifically, in industrial production, the probability of defe...
PUM
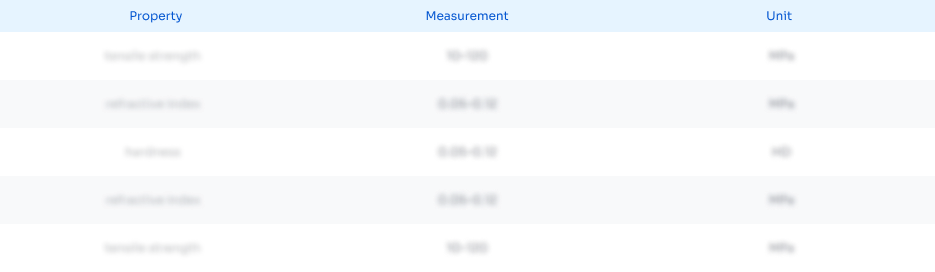
Abstract
Description
Claims
Application Information
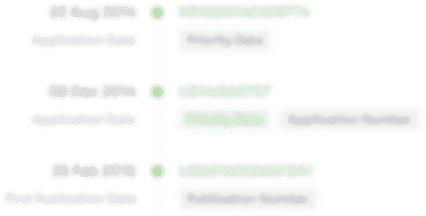
- R&D Engineer
- R&D Manager
- IP Professional
- Industry Leading Data Capabilities
- Powerful AI technology
- Patent DNA Extraction
Browse by: Latest US Patents, China's latest patents, Technical Efficacy Thesaurus, Application Domain, Technology Topic.
© 2024 PatSnap. All rights reserved.Legal|Privacy policy|Modern Slavery Act Transparency Statement|Sitemap