BERT anomaly detection method and equipment based on template sequence or word sequence
An anomaly detection and template sequence technology, applied in the field of log detection, can solve the problems that the anomaly detection model cannot achieve better detection results, achieve the effect of shortening training costs and improving the effect of anomaly detection
- Summary
- Abstract
- Description
- Claims
- Application Information
AI Technical Summary
Problems solved by technology
Method used
Image
Examples
no. 1 example ;
[0040] refer to Figure 1 to Figure 4 , an embodiment of the present invention provides a BERT anomaly detection method based on a word sequence, comprising the following steps:
[0041] Step S101, obtaining a plurality of original log messages;
[0042] Log messages are collected through plug-ins, such as log4net on the .net platform, and log4j and slf4j on the java platform. Such as figure 2 as shown, figure 2 9 original log messages are shown in the first block diagram from the left, for example: the third log message is "-1117848119 2005.06.03R16-M1-N2-C:J17-U012005-06-03-18.21.59.871925 R16-M1-N2-C: J17-U01 RAS KERNEL INFO CE SYM2, AT 0X0B85EEE0, MASK0X05".
[0043] Step S102, performing log analysis on each original log message, so as to obtain the log event corresponding to each original log message after analysis;
[0044] In this embodiment, the Drain log parsing tool is used to perform log parsing on all the obtained original log messages, and obtain the log e...
no. 2 example ;
[0067] refer to Figure 2 to Figure 5 , an embodiment of the present invention provides a BERT anomaly detection method based on a template sequence, comprising the following steps:
[0068] Step S201, obtaining a plurality of original log messages;
[0069] Step S202, performing log analysis on each original log message to obtain a log event corresponding to each original log message after analysis;
[0070] For the detailed introduction of step S201 and step S202, reference may be made to the first embodiment, and details are not repeated here.
[0071] Step S203, dividing all log events into a corresponding number of template sequences by using a window division method;
[0072] After the original semi-structured log messages are converted into structured log events, step S203 uses the fixed window technology to divide the logs into log sequences. It should be noted that, in this field, the concept of a log sequence is: to represent log messages in the same window. In t...
no. 3 example ;
[0084] refer to Figure 6 to Figure 8 , this embodiment uses the BGL data set generated in the BLUEGENE / L supercomputer system of LAWRENCE LIVERMORE NATIONAL LABS (LLNL). Table 2 below shows some basic information of the BGL dataset. The BGL dataset contains 4,747,963 original log messages, including 348,460 abnormal log messages. So the number of normal log messages is 4399503. All experiments are run on the GOOGLE COLAB cloud platform (HTTPS: / / COLAB.RESEARCH.GOOGLE.COM), which provides 8-core Online deep learning server with GOLD 6148CPU, TESLAK80 GPU and 25.51GB RAM. Three performance evaluation indicators commonly used in machine learning are used to evaluate the quality of the model, namely accuracy rate, recall rate, and F1-score.
[0085] system time span data size number of log messages exception message data BGL 7months 708M 4747963 348460
[0086] Table 2
[0087] In order to verify the rationality and advancement of the present...
PUM
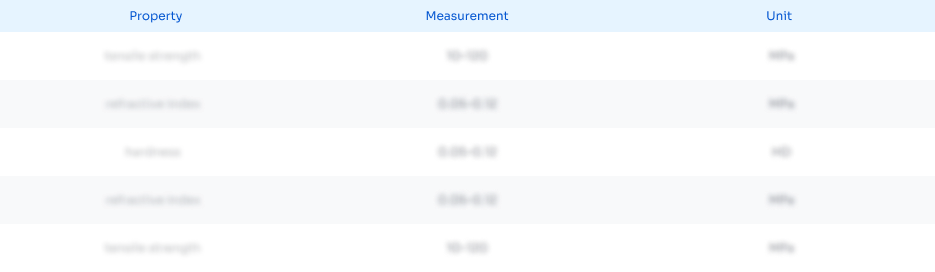
Abstract
Description
Claims
Application Information
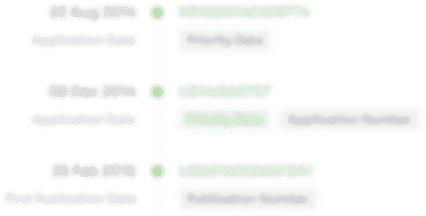
- R&D Engineer
- R&D Manager
- IP Professional
- Industry Leading Data Capabilities
- Powerful AI technology
- Patent DNA Extraction
Browse by: Latest US Patents, China's latest patents, Technical Efficacy Thesaurus, Application Domain, Technology Topic, Popular Technical Reports.
© 2024 PatSnap. All rights reserved.Legal|Privacy policy|Modern Slavery Act Transparency Statement|Sitemap|About US| Contact US: help@patsnap.com