Image zero-order classification model based on cross knowledge and classification method thereof
A sub-classification and image technology, applied in computer parts, character and pattern recognition, instruments, etc., can solve the problems of difficulty in discriminating models, discriminating unseen classes, reducing model performance, etc., to alleviate adverse effects, model and The method is simple and efficient, and the effect of increasing the intersection point
- Summary
- Abstract
- Description
- Claims
- Application Information
AI Technical Summary
Problems solved by technology
Method used
Image
Examples
Embodiment 1
[0089] A method for training an image zero-order classification model based on cross-knowledge, comprising the following steps:
[0090] Step S1: Construct a biological taxonomy tree according to all category names in the dataset;
[0091] Step S2: Input the image, text or attribute description in the data set into the visual feature extraction module and the semantic feature extraction module respectively, and extract the visual vector and semantic vector;
[0092] Step S3: According to the biological taxonomy tree and the visual vector, construct visual feature datasets at the Family level, Genus level, and Species level;
[0093] Step S4: According to the biological taxonomy tree and semantic vectors, construct semantic feature data sets at the Family level, Genus level, and Species level;
[0094] Step S5: Initialize the discriminator and the generator with classification regularization;
[0095] Step S6: Cross-select semantic features and visual features from the visual...
Embodiment 2
[0102] A method for training an image zero-order classification model based on cross-knowledge, comprising the following steps:
[0103] Step S1: Construct a biological taxonomy tree according to all category names in the dataset;
[0104] Step S2: Input the image, text or attribute description in the data set into the visual feature extraction module and the semantic feature extraction module respectively, and extract the visual vector and semantic vector;
[0105] Step S3: According to the biological taxonomy tree and the visual vector, construct visual feature datasets at the Family level, Genus level, and Species level;
[0106] Step S4: According to the biological taxonomy tree and semantic vectors, construct semantic feature data sets at the Family level, Genus level, and Species level;
[0107] Step S5: Initialize the discriminator and the generator with classification regularization;
[0108] Step S6: Cross-select semantic features and visual features from the visual...
Embodiment 3
[0115] A method for training an image zero-order classification model based on cross-knowledge, comprising the following steps:
[0116] Step S1: Construct a biological taxonomy tree according to all category names in the dataset;
[0117] Step S2: Input the image, text or attribute description in the data set into the visual feature extraction module and the semantic feature extraction module respectively, and extract the visual vector and semantic vector;
[0118] Step S3: According to the biological taxonomy tree and the visual vector, construct visual feature datasets at the Family level, Genus level, and Species level;
[0119] Step S4: According to the biological taxonomy tree and semantic vectors, construct semantic feature data sets at the Family level, Genus level, and Species level;
[0120] Step S5: Initialize the discriminator and the generator with classification regularization;
[0121] Step S6: Cross-select semantic features and visual features from the visual...
PUM
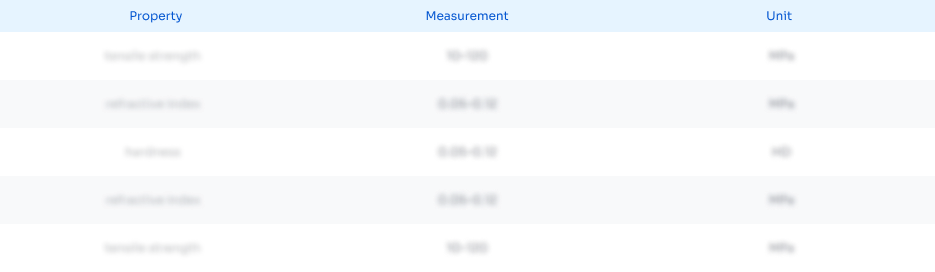
Abstract
Description
Claims
Application Information
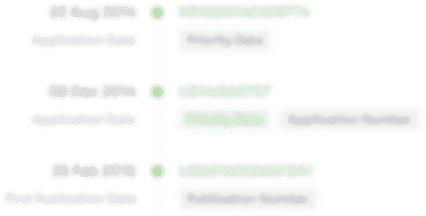
- Generate Ideas
- Intellectual Property
- Life Sciences
- Materials
- Tech Scout
- Unparalleled Data Quality
- Higher Quality Content
- 60% Fewer Hallucinations
Browse by: Latest US Patents, China's latest patents, Technical Efficacy Thesaurus, Application Domain, Technology Topic, Popular Technical Reports.
© 2025 PatSnap. All rights reserved.Legal|Privacy policy|Modern Slavery Act Transparency Statement|Sitemap|About US| Contact US: help@patsnap.com