Pedestrian re-identification implementation method based on active comparative learning
A technology for pedestrian re-identification and implementation method, applied in the field of pedestrian re-identification based on active contrastive learning, can solve the problem of high requirements for pedestrian fine-grained features, and achieve the effect of solving the problem of false labels
- Summary
- Abstract
- Description
- Claims
- Application Information
AI Technical Summary
Problems solved by technology
Method used
Image
Examples
Embodiment 1
[0042] like figure 1 , The present embodiment provides a comparison based on active learning heavy pedestrian identification implemented method of general process is as follows:
[0043] S1: First, based on a predicted loss by active learning module to select samples of high value, the number of each selected set of active learning is B t ;
[0044] S2: Select a pedestrian and then manually marking the sample via give pedestrians tag ID;
[0045] S3: The labeled sample is then defined into comparative sample learning module, with the same tag ID as the positive sample, the sample ID is marked as inconsistent definitions negative samples, and then optimize the contrast loss, characterized in that the same sample ID pedestrian distribution others close distance less; sample ID different distributions longer, the greater the distance;
[0046] S4: The test set weight input pedestrian recognition task completed training learning module contrast, for test results.
Embodiment 2
[0048] like figure 2 As shown in, for example, based on further refinement of the embodiment 1 of the present embodiment, there is provided a pedestrian based on active learning weight Comparative identify the particular method, comprising the steps of:
[0049] The S1, a high value to select the sample based on a predicted loss of active learning modules, specifically:
[0050] S1.1: The active learning module is broken down into modules and losses predicted target prediction modules. Wherein a plurality of intermediate target wherein the prediction module layer, an output layer and a layer composed Softmax, tag prediction for an unknown input data samples labeled pedestrian; loss prediction module by a plurality of functional layers, FC layers, wherein for the functional layer wherein the intermediate result of the processing target layer generated prediction module, means for generating the predicted loss.
[0051] S1.2: In active learning module, by the loss of the prediction ...
PUM
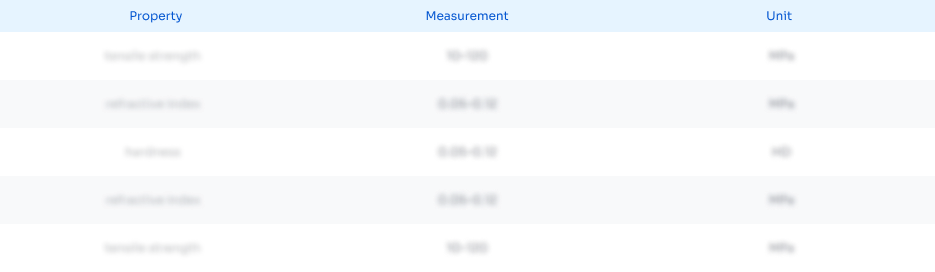
Abstract
Description
Claims
Application Information
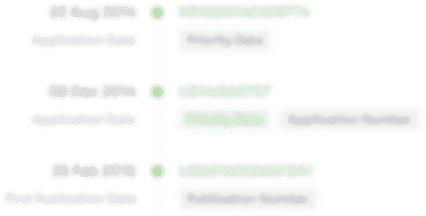
- R&D Engineer
- R&D Manager
- IP Professional
- Industry Leading Data Capabilities
- Powerful AI technology
- Patent DNA Extraction
Browse by: Latest US Patents, China's latest patents, Technical Efficacy Thesaurus, Application Domain, Technology Topic, Popular Technical Reports.
© 2024 PatSnap. All rights reserved.Legal|Privacy policy|Modern Slavery Act Transparency Statement|Sitemap|About US| Contact US: help@patsnap.com