Generalized zero sample target classification method based on active learning and variational auto-encoder
A self-encoder, active learning technology, applied in instruments, computer parts, character and pattern recognition, etc., can solve the problem of low accuracy of generalized zero-sample target classification, reduce difficulty, improve accuracy, and eliminate bias. effect of the problem
- Summary
- Abstract
- Description
- Claims
- Application Information
AI Technical Summary
Problems solved by technology
Method used
Image
Examples
Embodiment Construction
[0045] The present invention will be further described in detail below in conjunction with the accompanying drawings and specific embodiments.
[0046] Step 1, get the training sample set P train and the test sample set P test :
[0047] will be obtained from the zero-shot image set O containing n s n of known target classes 1 A known class training sample set P consisting of images and the target class label of each known class image train s , and obtained from O containing n u n of unknown target classes 2 images to form the unknown class training sample set P train u , forming the training sample set P train , and at the same time will contain n obtained from O u m images of unknown target categories form the test sample set P test , in this embodiment, the zero sample image set O is the AWA1 data set, n s =40,n 1 = 17060, n u =10,n 2 =4251, m=9164, and satisfy n 1 +n 2 >m;
[0048] Step 2, construct a generalized zero-shot object classification model H ba...
PUM
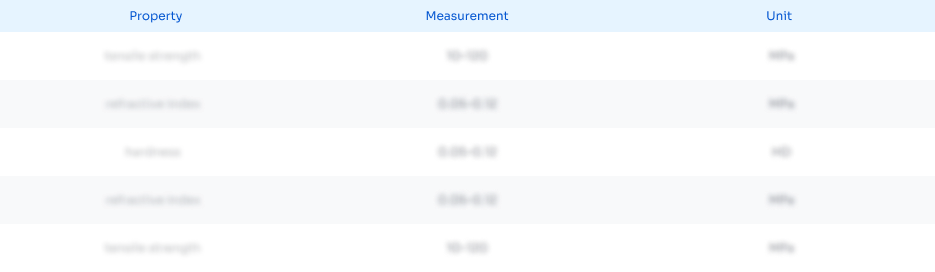
Abstract
Description
Claims
Application Information
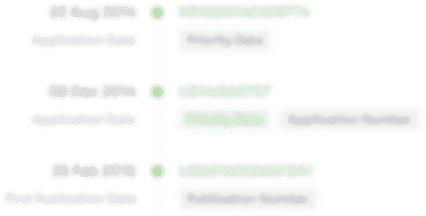
- R&D Engineer
- R&D Manager
- IP Professional
- Industry Leading Data Capabilities
- Powerful AI technology
- Patent DNA Extraction
Browse by: Latest US Patents, China's latest patents, Technical Efficacy Thesaurus, Application Domain, Technology Topic, Popular Technical Reports.
© 2024 PatSnap. All rights reserved.Legal|Privacy policy|Modern Slavery Act Transparency Statement|Sitemap|About US| Contact US: help@patsnap.com