Automatic segmentation method for rectal cancer CT image based on U-Transformer
A CT image and automatic segmentation technology, applied in image analysis, neural learning methods, image data processing, etc., can solve problems such as instability and low efficiency of manual segmentation, achieve high-precision medical segmentation, realize medical segmentation, and avoid low efficiency. and unstable effects
- Summary
- Abstract
- Description
- Claims
- Application Information
AI Technical Summary
Problems solved by technology
Method used
Image
Examples
Embodiment 1
[0085] Embodiment 1: Data preprocessing module: preprocessing to experimental data
[0086] (1) Perform histogram equalization and normalization processing on the data, such as figure 1 shown.
[0087] (2) Carry out data regulation on CT images and build a protocol database.
[0088] (3) Carry out geometric transformation methods such as rotation, mirror image, and horizontal flip on CT images, and perform data enhancement and amplify training samples to reduce overfitting.
[0089] (4) Unify the size of each CT image and marker map.
[0090] (5) Divide the data set into training set, verification set and test set according to the ratio of 0.8:0.1:0.1.
Embodiment 2
[0091] Embodiment 2: U-Transformer network model construction module: construct U-Transformer network model.
[0092] (1) Construct the Patch Embedding layer. Transform the two-dimensional CT image to obtain M pieces of size P 2 One-dimensional patch embeddings of C.
[0093] (2) Build the Swin Transformer Block. The specific Swin Transformer Block such as Figure 4 Shown: 1. Use the W-MSA mechanism to calculate the self-attention score inside the window 2. Use the SW-MSA mechanism to calculate the attention score between different windows.
[0094] (3) Build Patch Merging. The specific Patch Merging performs interval sampling on the H and W dimensions and stitches them together to achieve the purpose of downsampling.
[0095] (4) Construct full-scale skip connections. The specific full-scale skip connection mechanism is as follows: image 3 Shown: 1. For high-level semantic features, we first use maximum pooling to reduce the size of its feature map, and then use a 3×3...
Embodiment 3
[0098] Embodiment 3: U-Transformer network model training module: training U-Transformer network model
[0099] (1) The Adam optimization method is used as the optimization method, and the cross entropy is used as the loss function for training. The cross-entropy formula is as follows:
[0100]
[0101] Among them, y i is the value of the pixel point i in the real eye mask diagram of the table note, and the value is 0 or 1; is the value of pixel i in the mask image obtained by the algorithm, and the value range is 0 to 1; N is the total number of pixels in the segmentation image and the annotation mask image.
[0102] (2) Pre-train the U-Transformer network model on the CIFAR-100 dataset.
PUM
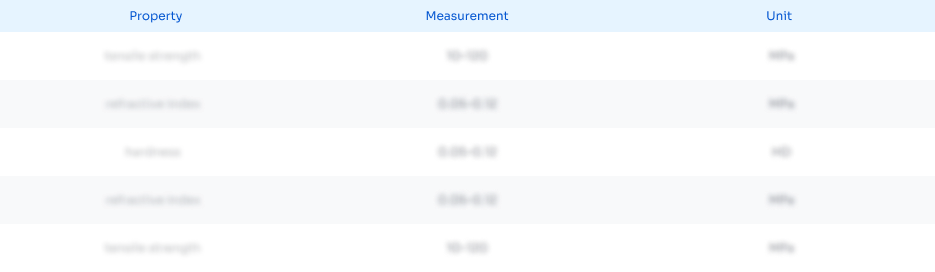
Abstract
Description
Claims
Application Information
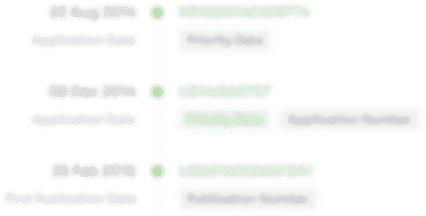
- R&D Engineer
- R&D Manager
- IP Professional
- Industry Leading Data Capabilities
- Powerful AI technology
- Patent DNA Extraction
Browse by: Latest US Patents, China's latest patents, Technical Efficacy Thesaurus, Application Domain, Technology Topic, Popular Technical Reports.
© 2024 PatSnap. All rights reserved.Legal|Privacy policy|Modern Slavery Act Transparency Statement|Sitemap|About US| Contact US: help@patsnap.com