Supervised dictionary learning audio classification method and system based on data driving, and medium
A dictionary learning, data-driven technology, applied in the field of sparse representation, to achieve the effect of improving pairwise orthogonality, excellent generalization ability, and excellent performance
- Summary
- Abstract
- Description
- Claims
- Application Information
AI Technical Summary
Benefits of technology
Problems solved by technology
Method used
Image
Examples
Embodiment
[0050] Such as figure 1 As shown, the present embodiment provides a data-driven supervised dictionary learning audio classification method, comprising the following steps:
[0051] S1. Determine the number of categories C of the sample set, and use the input sample x n , and its corresponding class label y n Train C class-specific dictionaries D c , c∈[1,C], such as figure 2 As shown, it specifically includes the following steps:
[0052] S11. Initialize dictionary D c 0 , learning rate η 0 , the learning rate update rate α, the number of iterations T;
[0053] S12. Determine the loss function J;
[0054] Furthermore, the specific form of the loss function J is:
[0055] J(A,D)=J 1 (D, A)+μJ 2 (D, A)+λJ 3 (A)+γ 1 J 4 (A)+γ 2 J 5 (D);
[0056]
[0057]
[0058]
[0059]
[0060]
[0061] Among them, μ is the sample constraint parameter, λ is the classifier constraint parameter, γ 1 Constraint parameter for sparse coding, γ 2 Learn constraint p...
PUM
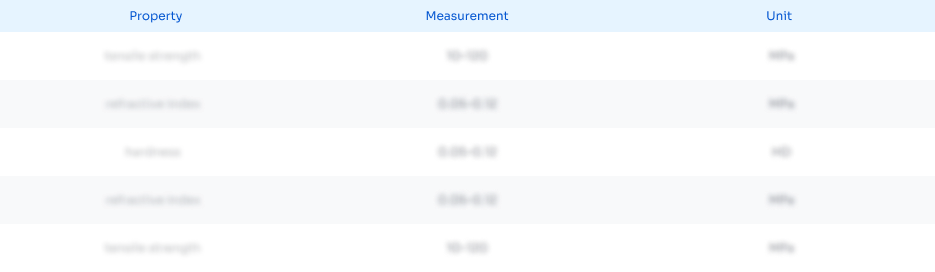
Abstract
Description
Claims
Application Information
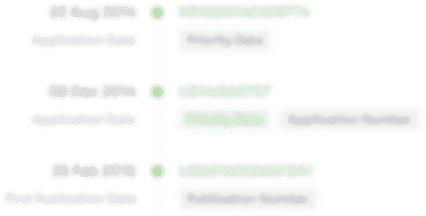
- R&D
- Intellectual Property
- Life Sciences
- Materials
- Tech Scout
- Unparalleled Data Quality
- Higher Quality Content
- 60% Fewer Hallucinations
Browse by: Latest US Patents, China's latest patents, Technical Efficacy Thesaurus, Application Domain, Technology Topic, Popular Technical Reports.
© 2025 PatSnap. All rights reserved.Legal|Privacy policy|Modern Slavery Act Transparency Statement|Sitemap|About US| Contact US: help@patsnap.com