Fractional-order neural network modeling method based on smooth Group Lasso penalty term
A neural network modeling and neural network model technology, applied in the field of neural network model modeling, can solve the problems of not getting the global optimal solution, slow convergence speed, complex neural network structure, etc., to improve the network generalization ability, Simplified network structure and good sparsity
- Summary
- Abstract
- Description
- Claims
- Application Information
AI Technical Summary
Problems solved by technology
Method used
Image
Examples
Embodiment Construction
[0044] The present invention will be further described below in conjunction with the accompanying drawings and embodiments.
[0045] Such asfigure 1 As shown, the fractional order neural network modeling method based on the smooth Group Lasso penalty term includes the following steps:
[0046] Step 1, select the neural network model, use the smooth function to approximate the Group Lasso penalty term, and obtain the error function;
[0047] Step 2, using the fractional steepest descent method to train the network weights, that is, the weights are updated along the direction of the fractional negative gradient of the error function with respect to the weights;
[0048] Step 3: Obtain the network parameters of the neural network model according to the fractional steepest descent method in step 2;
[0049] Step 4: Use the test samples to calculate the accuracy of the neural network model.
[0050] Preferably, the neural network model of said step 1 is as follows:
[0051] Sele...
PUM
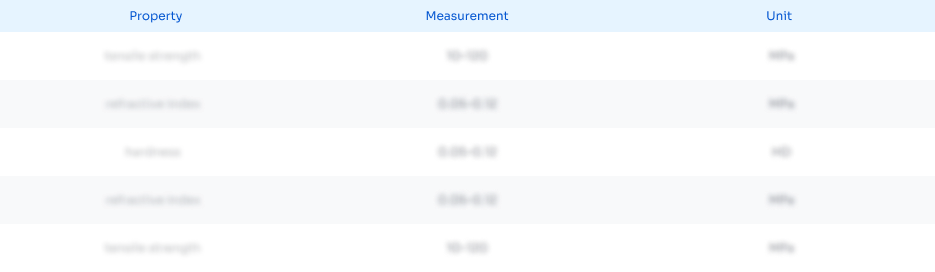
Abstract
Description
Claims
Application Information
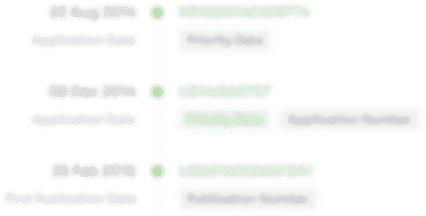
- R&D
- Intellectual Property
- Life Sciences
- Materials
- Tech Scout
- Unparalleled Data Quality
- Higher Quality Content
- 60% Fewer Hallucinations
Browse by: Latest US Patents, China's latest patents, Technical Efficacy Thesaurus, Application Domain, Technology Topic, Popular Technical Reports.
© 2025 PatSnap. All rights reserved.Legal|Privacy policy|Modern Slavery Act Transparency Statement|Sitemap|About US| Contact US: help@patsnap.com