A Few-Shot Object Classification Method Based on Two-way Structural Contrastive Embedding Learning
A classification method and small sample technology, applied in the field of computer vision, can solve problems such as long training time and multiple computing resources, and achieve the effect of simple implementation method and improved generalization ability.
- Summary
- Abstract
- Description
- Claims
- Application Information
AI Technical Summary
Problems solved by technology
Method used
Image
Examples
Embodiment Construction
[0035] In order to make the purpose, technical solution and technical effect of the present invention clearer, the present invention will be further described in detail below in conjunction with the accompanying drawings.
[0036] The present invention uses large-scale labeled training data of the basic class to construct a classifier based on a deep convolutional network in a supervised manner, and uses the classifier as a teacher path to guide the learning of feature embedding in the student path, and randomly extracts batches from the basic class The image is data enhanced, and the enhanced image is used for structured contrastive embedding learning. By adding structural similarity in the comparative embedding learning process, the learned feature embedding learning network is more generalizable. On this basis, for a new object category, first use a small amount of labeled data in each category to calculate the category prototype, then calculate the similarity between the te...
PUM
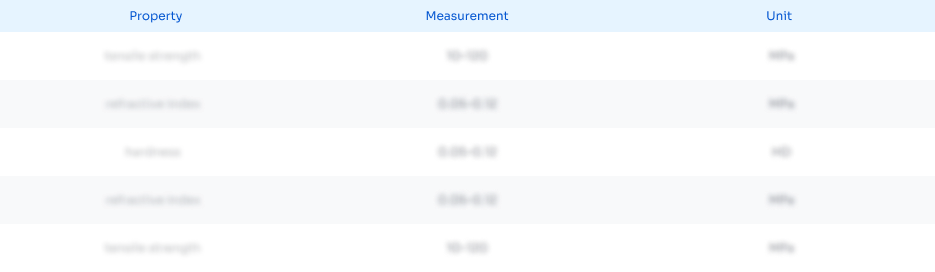
Abstract
Description
Claims
Application Information
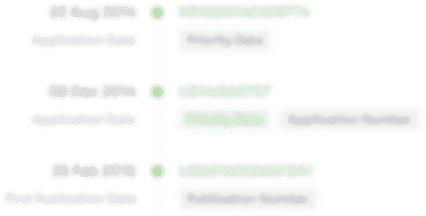
- R&D
- Intellectual Property
- Life Sciences
- Materials
- Tech Scout
- Unparalleled Data Quality
- Higher Quality Content
- 60% Fewer Hallucinations
Browse by: Latest US Patents, China's latest patents, Technical Efficacy Thesaurus, Application Domain, Technology Topic, Popular Technical Reports.
© 2025 PatSnap. All rights reserved.Legal|Privacy policy|Modern Slavery Act Transparency Statement|Sitemap|About US| Contact US: help@patsnap.com