Feature space reformation-based teacher-student architecture noise-tag-containing image classification method
A technology of feature space and classification method, applied in neural architecture, neural learning method, biological neural network model, etc., can solve the problem of ignoring the benefits of mutual learning, to promote self-learning, improve performance, robustness and effectiveness excellent effect
- Summary
- Abstract
- Description
- Claims
- Application Information
AI Technical Summary
Problems solved by technology
Method used
Image
Examples
Embodiment Construction
[0027] 1. Related work
[0028] 1.1 Noise Label Learning
[0029]Due to its strong learning ability, deep neural networks can easily adapt to any noise. Training a deep neural network on a noisy data set allows the network to fit noise samples, which greatly reduces the generalization ability of the model. To overcome this problem, some researchers modify the loss function to make the model more robust to noise. This approach has strong theoretical support, but as the complexity of the noise increases, the effectiveness of the approach gradually decreases. At the same time, the modification of the loss function increases the amount of computation required for training convergence. Since learning noisy labels directly cannot achieve good results, researchers instead consider correcting noisy labels. Reed et al. [Reed, Scott, et al. "Training deep neural networks on noisy labels with bootstrapping." arXiv preprint arXiv:1412.6596 (2014).] combine raw labels with model predic...
PUM
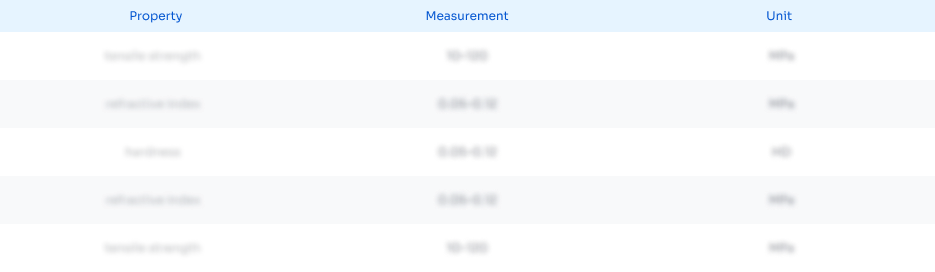
Abstract
Description
Claims
Application Information
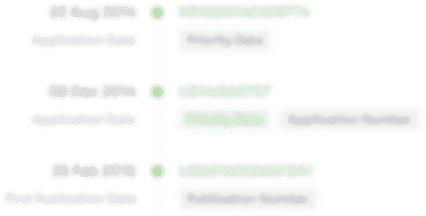
- R&D Engineer
- R&D Manager
- IP Professional
- Industry Leading Data Capabilities
- Powerful AI technology
- Patent DNA Extraction
Browse by: Latest US Patents, China's latest patents, Technical Efficacy Thesaurus, Application Domain, Technology Topic, Popular Technical Reports.
© 2024 PatSnap. All rights reserved.Legal|Privacy policy|Modern Slavery Act Transparency Statement|Sitemap|About US| Contact US: help@patsnap.com