Keyword vectorization method based on topic semantic information and application thereof
- Summary
- Abstract
- Description
- Claims
- Application Information
AI Technical Summary
Problems solved by technology
Method used
Image
Examples
Embodiment Construction
[0044] The present invention will be described in further detail below in conjunction with the accompanying drawings.
[0045] figure 1 It is a flowchart of the present invention, describing the process of keyword vectorization based on subject semantic information. For the convenience of description, the following specific example is given. This example mainly solves the problem of document retrieval. It is based on the 20newsgroups data set, which contains 20 different categories of news and a total of 11315 articles. The relevant symbols are defined as follows:
[0046] document set D = {d 1 , d 2 ,...,d n}, remove stop words and extract keywords from each document in the document set D to form a keyword set W={w 1 ,w 2 ,...,w u}, the topic set obtained by HDBSCAN clustering algorithm is T={t 1 ,t 2 ,...,t m}. is the document vector matrix trained by the Sentence-BERT model on the document set D. is the reduced document vector matrix output by the UMAP dimensi...
PUM
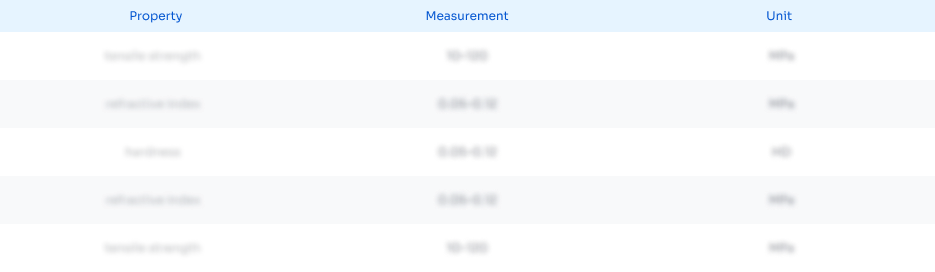
Abstract
Description
Claims
Application Information
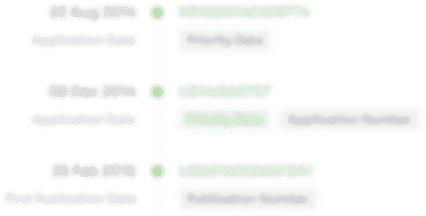
- R&D Engineer
- R&D Manager
- IP Professional
- Industry Leading Data Capabilities
- Powerful AI technology
- Patent DNA Extraction
Browse by: Latest US Patents, China's latest patents, Technical Efficacy Thesaurus, Application Domain, Technology Topic, Popular Technical Reports.
© 2024 PatSnap. All rights reserved.Legal|Privacy policy|Modern Slavery Act Transparency Statement|Sitemap|About US| Contact US: help@patsnap.com