Multi-source manifold electroencephalogram feature transfer learning method
A technology of transfer learning and EEG, applied in the research field of nervous system motion control mechanism, can solve problems such as large amount of calculation and incompatibility of machine learning methods, and achieve the effect of avoiding weight
- Summary
- Abstract
- Description
- Claims
- Application Information
AI Technical Summary
Problems solved by technology
Method used
Image
Examples
Embodiment Construction
[0078] The embodiments of the present invention will be described in detail below in conjunction with the accompanying drawings: this embodiment is implemented on the premise of the technical solution of the present invention, and provides a detailed implementation plan and a specific operation process.
[0079] Due to the traditional single-source EEG signal migration, there is a problem that the performance is unstable with different source domain transferability. The invention proposes a multi-source manifold EEG feature transfer learning method. Such as figure 1 As shown, the implementation of the present invention mainly includes six steps: (1) EEG manifold feature extraction; (2) manifold feature migration; (3) multi-source migration framework.
[0080] Each step will be described in detail below one by one.
[0081] Step 1: EEG feature extraction
[0082] Write down the covariance matrix of the EEG signal of an experiment as P, P=XX T , and P is the SPD matrix. use...
PUM
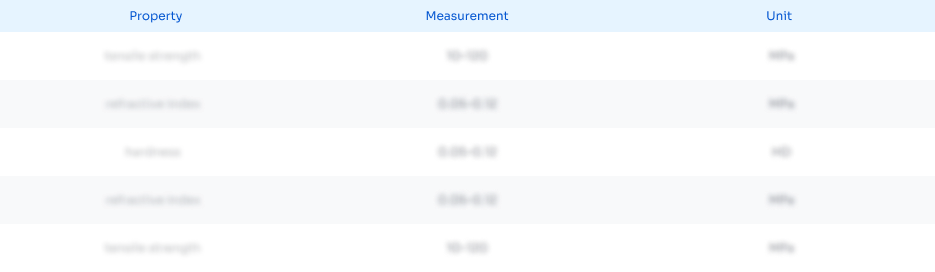
Abstract
Description
Claims
Application Information
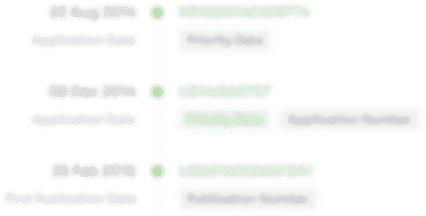
- R&D Engineer
- R&D Manager
- IP Professional
- Industry Leading Data Capabilities
- Powerful AI technology
- Patent DNA Extraction
Browse by: Latest US Patents, China's latest patents, Technical Efficacy Thesaurus, Application Domain, Technology Topic, Popular Technical Reports.
© 2024 PatSnap. All rights reserved.Legal|Privacy policy|Modern Slavery Act Transparency Statement|Sitemap|About US| Contact US: help@patsnap.com