Retinopathy detection system based on lesion attention pyramid convolutional neural network
A convolutional neural network and retinopathy technology, applied in the field of medical image processing, can solve the problems of low detection accuracy of retinopathy, weak interpretability, inability to use multi-resolution image information, etc., and achieve good retinopathy diagnosis performance, The effect of improving accuracy
- Summary
- Abstract
- Description
- Claims
- Application Information
AI Technical Summary
Problems solved by technology
Method used
Image
Examples
specific Embodiment approach 1
[0027] Specific Embodiment 1: In this embodiment, the retinal lesion detection system based on the lesion-focused pyramid convolutional neural network includes:
[0028] Image processing main module, neural network main module, training main module, detection main module;
[0029] The image processing main module is used to collect original retinopathy images, preprocess the collected original retinopathy images, and obtain preprocessed original retinopathy images;
[0030] The main module of the neural network is used to build a lesion-focused pyramid convolutional neural network model;
[0031] The training main module uses the preprocessed original retinal lesion image to train the built lesion-focused pyramid convolutional neural network model, and obtains the trained lesion-focused pyramidal convolutional neural network model;
[0032] The detection main module is used to load a trained lesion-focused pyramid convolutional neural network model to classify images of retin...
specific Embodiment approach 2
[0033] Specific embodiment 2: The difference between this embodiment and specific embodiment 1 is that the image processing main module is used to collect the original retinopathy image, preprocess the collected original retinopathy image, and obtain the preprocessed original retinopathy image; the specific process is:
[0034] Step A1. Preprocessing the original retinopathy image to construct a multi-resolution image; the specific process is:
[0035] Obtain the original retinopathy image set, resize the resolution of each image into a set of multi-resolution images of 1024×1024, 512×512 and 256×256;
[0036] There are some background areas around the retinal images that do not affect the classification results, so each group of multi-resolution images of 1024×1024, 512×512 and 256×256 is cropped to 896×896, 448×448 and 224×224 at the center of the image Multi-resolution images (1024×1024 cropped to 896×896, 512×512 cropped to 448×448, 256×256 cropped to 224×224), each group...
specific Embodiment approach 3
[0043] Specific embodiment three: the difference between this embodiment and specific embodiment one or two is that the main module of the neural network is used to build a lesion-focused pyramid convolutional neural network model; the specific process is:
[0044] The lesion-focused pyramid convolutional neural network model includes a low-resolution network, a medium-resolution network, a high-resolution network, and a weakly supervised localization module;
[0045] B1. The low-resolution network includes a basic module 1, a basic module 3, a basic module 4, a basic module 5, a basic module 6 and a basic module 7 in sequence;
[0046] The medium-resolution network includes a basic module 1, a basic module 3 and a basic module 4 in sequence;
[0047] The high-resolution network sequentially includes a basic module 1, a basic module 2, a basic module 3, a basic module 4, a basic module 5, a basic module 6 and a basic module 7;
[0048] The basic module 1 sequentially includes...
PUM
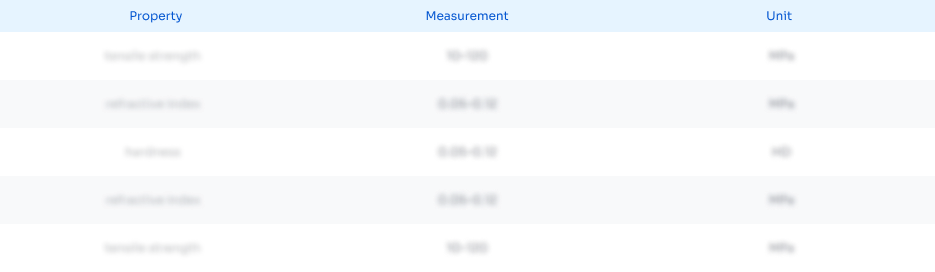
Abstract
Description
Claims
Application Information
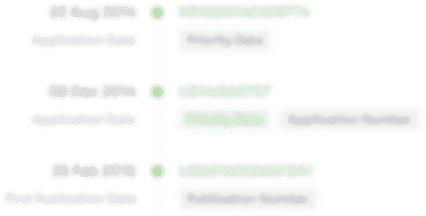
- Generate Ideas
- Intellectual Property
- Life Sciences
- Materials
- Tech Scout
- Unparalleled Data Quality
- Higher Quality Content
- 60% Fewer Hallucinations
Browse by: Latest US Patents, China's latest patents, Technical Efficacy Thesaurus, Application Domain, Technology Topic, Popular Technical Reports.
© 2025 PatSnap. All rights reserved.Legal|Privacy policy|Modern Slavery Act Transparency Statement|Sitemap|About US| Contact US: help@patsnap.com