Mitigating adversarial effects in machine learning systems
A machine learning and adversarial technology, applied in the field of machine learning, can solve problems such as impossible detection and difficult detection of resistance, and achieve the effect of maintaining accuracy and fidelity, reducing risks, and minimizing risks
- Summary
- Abstract
- Description
- Claims
- Application Information
AI Technical Summary
Problems solved by technology
Method used
Image
Examples
Embodiment Construction
[0023] Embodiments of the present disclosure provide techniques for sanitizing and / or repairing machine learning (ML) models in order to mitigate adversarial attacks. As used herein, adversarial data and adversarial models generally refer to data or models that are close to legitimate (or appear legitimate) in some spaces but exhibit unwanted or malicious behavior in other spaces. For example, an adversarial model can provide accurate and desired results with a certain set of input data. However, a model is adversarial if it contains some internal weights or biases that make it respond to some inputs in an adversarial or undesired way. For example, an infected or poisoned model may return incorrect results when certain triggers are present in the input. In many embodiments, these triggers may include patterns in the input data. Often, these triggers are hidden in the input data and are imperceptible to human observers.
[0024] An example of an adversarial model is a model ...
PUM
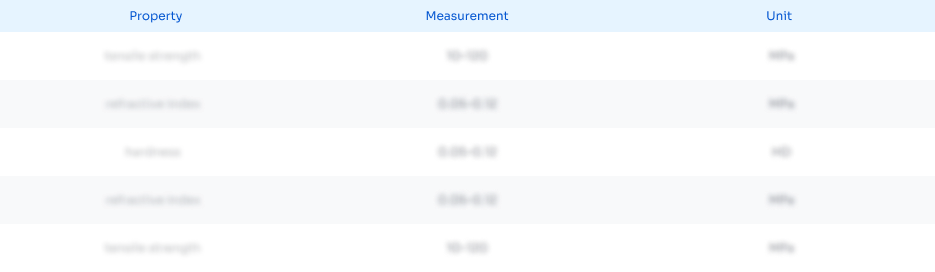
Abstract
Description
Claims
Application Information
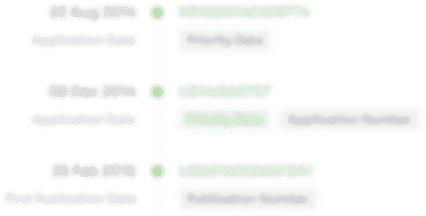
- R&D Engineer
- R&D Manager
- IP Professional
- Industry Leading Data Capabilities
- Powerful AI technology
- Patent DNA Extraction
Browse by: Latest US Patents, China's latest patents, Technical Efficacy Thesaurus, Application Domain, Technology Topic, Popular Technical Reports.
© 2024 PatSnap. All rights reserved.Legal|Privacy policy|Modern Slavery Act Transparency Statement|Sitemap|About US| Contact US: help@patsnap.com