Machine supervised learning method and device
A technology of supervised learning and machine learning models, applied in the field of machine supervised learning methods and devices, can solve the problems of uncontrollable training process and uncorrectable machine learning models, etc., and achieve the effect of accurate results
- Summary
- Abstract
- Description
- Claims
- Application Information
AI Technical Summary
Problems solved by technology
Method used
Image
Examples
no. 1 approach
[0032] see figure 1 , the first embodiment of the machine supervised learning method of the present invention comprises the following steps:
[0033] Step S1: Manually judge multiple classification results of the machine learning model to create a black sample set and a white sample set.
[0034] Each sample data in the black sample set and the white sample set corresponds to a feature vector, and each feature vector includes multiple feature dimensions.
[0035] For example, machine supervised learning is used to predict and classify the behavior of the dialogue bill to determine whether the bill is a normal bill or an abnormal bill such as fraud. The black sample set includes, for example, multiple sample data that have been manually judged as abnormal bills, and the white sample set. For example, it includes a plurality of sample data that are judged to be normal by manual research.
[0036] Step S2: Perform sample collision on the black sample set and the white sample se...
no. 2 approach
[0041] see figure 2 , the second embodiment of the machine supervised learning method of the present invention comprises the following steps:
[0042] Step S1a: use the training sample set to perform machine learning to obtain a machine learning model.
[0043] Step S1b: Use the machine learning model to predict and classify the test sample set to obtain multiple classification results.
[0044] Step S1: Manually judge multiple classification results of the machine learning model to create a black sample set and a white sample set.
[0045] It should be understood that step S1 is to manually study and judge the multiple classification results of step S1b.
[0046] Step S2a: Manually set the corresponding machine weights for each feature dimension of the feature vector.
[0047] It should be understood that the initial machine weights of each feature dimension can be set through step S2a. For example, one sample data of the black sample set corresponds to a feature vector ...
PUM
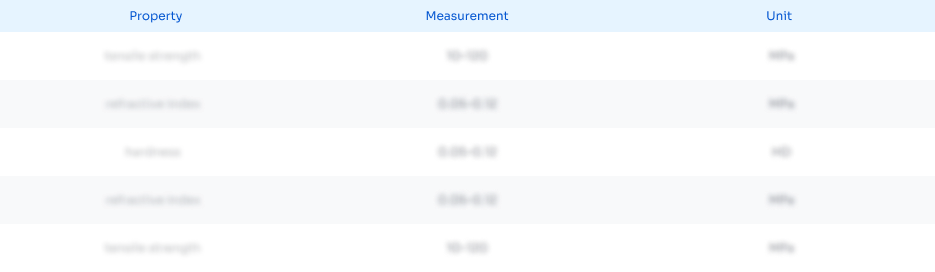
Abstract
Description
Claims
Application Information
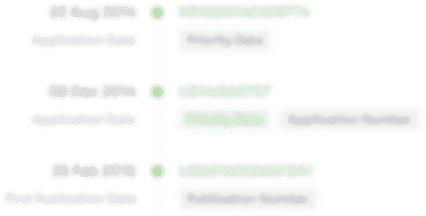
- R&D
- Intellectual Property
- Life Sciences
- Materials
- Tech Scout
- Unparalleled Data Quality
- Higher Quality Content
- 60% Fewer Hallucinations
Browse by: Latest US Patents, China's latest patents, Technical Efficacy Thesaurus, Application Domain, Technology Topic, Popular Technical Reports.
© 2025 PatSnap. All rights reserved.Legal|Privacy policy|Modern Slavery Act Transparency Statement|Sitemap|About US| Contact US: help@patsnap.com