Deep attention embedded graph clustering method with smooth structure
An attention and graph clustering technology, applied in the field of graph data processing, can solve problems such as clustering performance degradation, achieve the effect of eliminating instability and solving performance degradation problems
- Summary
- Abstract
- Description
- Claims
- Application Information
AI Technical Summary
Problems solved by technology
Method used
Image
Examples
Embodiment
[0033] like figure 1 The implementation process of the deep attention embedded graph clustering method with smooth structure provided by the embodiment of the present invention is shown. For convenience of description, only the part related to the embodiment of the present invention is shown. Details are as follows:
[0034] Step 1. Preprocess the graph data, construct the basic attribute graph G=(V, E, X, A), and set the structure of the basic graph attention coding network, including the number of network layers, the dimension of the hidden layer, Output dimension and initial clustering accuracy Acc;
[0035] Step 2. Input the graph structure and node attribute information to define the loss term L of the graph coding network r with L s , some hyperparameters are pre-set by a multi-group cross-validation method, including the penalty term coefficient: ρ l with λ l ;
[0036] Step 3. Integrate the structural reconstruction loss L r and the smoothness constraint L s , ...
PUM
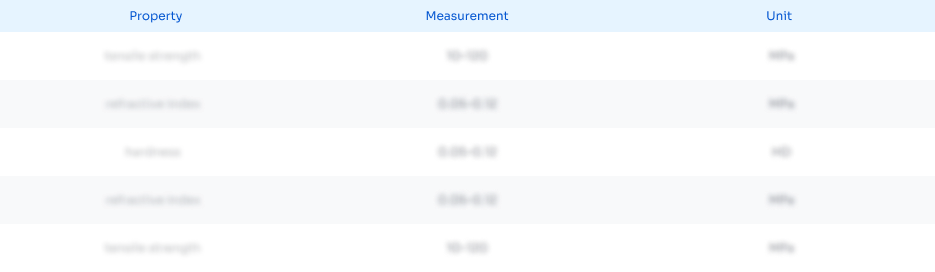
Abstract
Description
Claims
Application Information
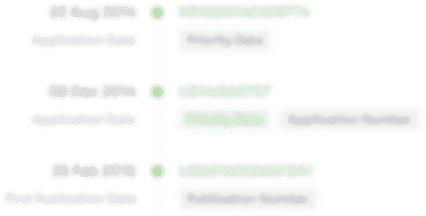
- R&D Engineer
- R&D Manager
- IP Professional
- Industry Leading Data Capabilities
- Powerful AI technology
- Patent DNA Extraction
Browse by: Latest US Patents, China's latest patents, Technical Efficacy Thesaurus, Application Domain, Technology Topic, Popular Technical Reports.
© 2024 PatSnap. All rights reserved.Legal|Privacy policy|Modern Slavery Act Transparency Statement|Sitemap|About US| Contact US: help@patsnap.com