Method of evaluating learning rate of recommender systems
a recommendation system and learning rate technology, applied in the field of evaluating the learning rate of recommender systems, can solve the problems of not being completely neutral in the recommendation algorithm, affecting the reliability and reputation of the recommendation system, and not being able to recommend items, etc., and achieve the effect of improving online advertising campaigns
- Summary
- Abstract
- Description
- Claims
- Application Information
AI Technical Summary
Benefits of technology
Problems solved by technology
Method used
Image
Examples
Embodiment Construction
[0040]As noted above, a content provider may use the present process to test, monitor and report on the performance of a content service provider, including a recommender system employed by the latter, to see if it is behaving in accordance with a particular policy, and / or if it is showing some measurable bias. A “recommender system” in this instance refers to a type of intelligent software agent which tailors a recommendation or suggestion for an item to a particular subscriber, based on characteristics of the subscriber, the item itself, or some combination thereof. In other words, a recommender system may incorporate some randomization features, but does not operate entirely based on a “random” presentation of content to a subscriber, or on a purely “programmed” presentation of content. Thus, a recommender system typically bases a particular recommendation to a particular subscriber based on explicit and implicit data obtained from such subscriber. The latter, of course, can incl...
PUM
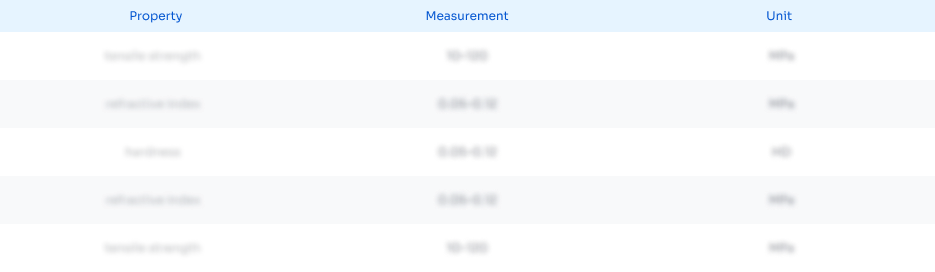
Abstract
Description
Claims
Application Information
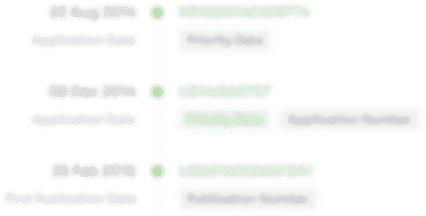
- R&D Engineer
- R&D Manager
- IP Professional
- Industry Leading Data Capabilities
- Powerful AI technology
- Patent DNA Extraction
Browse by: Latest US Patents, China's latest patents, Technical Efficacy Thesaurus, Application Domain, Technology Topic, Popular Technical Reports.
© 2024 PatSnap. All rights reserved.Legal|Privacy policy|Modern Slavery Act Transparency Statement|Sitemap|About US| Contact US: help@patsnap.com