A Genetic Fuzzy Clustering Image Segmentation Method
A technology of fuzzy clustering and image segmentation, which is applied in the field of genetic fuzzy clustering image segmentation, can solve problems affecting the accuracy of segmentation, generating noise in segmentation results, and interfering with the selection of clustering centers by algorithms
- Summary
- Abstract
- Description
- Claims
- Application Information
AI Technical Summary
Problems solved by technology
Method used
Image
Examples
example
[0094] Artificially simulated images with a size of 300×300 were added with Gaussian noise with a mean of 0 and a variance of 0.005.
[0095] In step (1.1), λ=3, L=5, μ=0.7, and the neighborhood R takes a 3×3 rectangular window;
[0096] In step (2.1), population size Q=20, N c = 4;
[0097] In step (2.2), m=2, MinD=20, α=1000000, β=4000;
[0098] In step (2.3), D=1, the cross ratio is 50%, and the variation ratio is 5%;
[0099] In step (2.5), M=3, T=30.
PUM
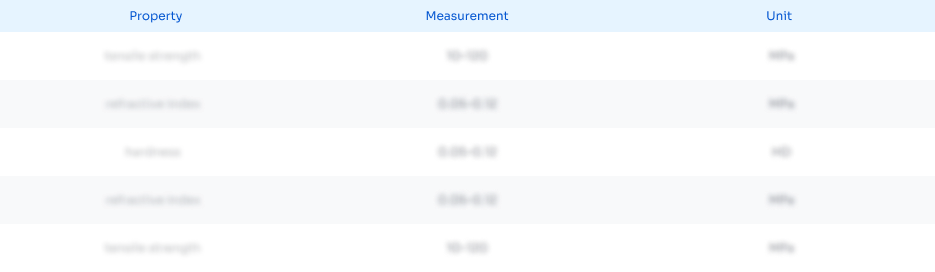
Abstract
Description
Claims
Application Information
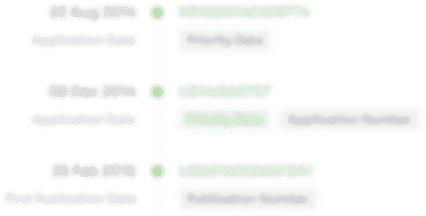
- R&D Engineer
- R&D Manager
- IP Professional
- Industry Leading Data Capabilities
- Powerful AI technology
- Patent DNA Extraction
Browse by: Latest US Patents, China's latest patents, Technical Efficacy Thesaurus, Application Domain, Technology Topic, Popular Technical Reports.
© 2024 PatSnap. All rights reserved.Legal|Privacy policy|Modern Slavery Act Transparency Statement|Sitemap|About US| Contact US: help@patsnap.com