Multiclass image classification method based on active learning and semi-supervised learning
A semi-supervised learning and active learning technology, applied in character and pattern recognition, instruments, calculations, etc., can solve the problems of waste of useful information, failure to describe the uncertainty of sample classification well, and failure to consider information, etc., to achieve rapid provision of , efficient image classification effect, and the effect of taking into account the requirements of computational complexity
- Summary
- Abstract
- Description
- Claims
- Application Information
AI Technical Summary
Problems solved by technology
Method used
Image
Examples
Embodiment Construction
[0029] The core of the multi-class image classification method based on active learning and semi-supervised learning proposed by the present invention mainly includes three parts: active learning based on optimal and suboptimal labels (BvSB), self-training (CST) semi-analysis with constraints Supervised learning, and support vector machine (SVM) classifiers.
[0030] Support vector machine (SVM) classifiers are well known to those skilled in the art.
[0031] The active learning method based on optimal label and suboptimal label (BvSB) is one of the uncertainty sampling methods. In multi-class classification tasks, it can effectively select the samples with the highest classification uncertainty for the current classifier, so that better classification performance can be obtained with fewer training samples. The basic block diagram of BvSB active learning can be found in the appendix figure 1 .
[0032] Self-training (Self-Training) is a technique commonly used in semi-supe...
PUM
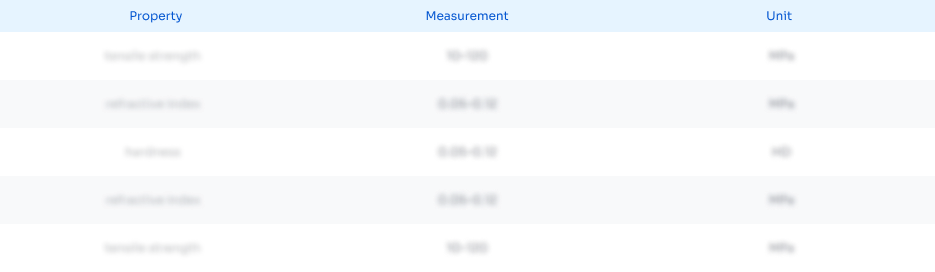
Abstract
Description
Claims
Application Information
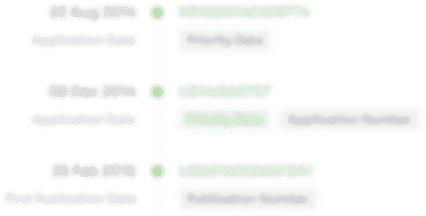
- R&D Engineer
- R&D Manager
- IP Professional
- Industry Leading Data Capabilities
- Powerful AI technology
- Patent DNA Extraction
Browse by: Latest US Patents, China's latest patents, Technical Efficacy Thesaurus, Application Domain, Technology Topic, Popular Technical Reports.
© 2024 PatSnap. All rights reserved.Legal|Privacy policy|Modern Slavery Act Transparency Statement|Sitemap|About US| Contact US: help@patsnap.com