Image marking method based on characteristic scene
A feature scene, image annotation technology, applied in special data processing applications, instruments, electronic digital data processing and other directions, can solve the problem of inability to guarantee semantic content consistency, to ensure semantic consistency, increase consistency, and improve reliability. Effect
- Summary
- Abstract
- Description
- Claims
- Application Information
AI Technical Summary
Problems solved by technology
Method used
Image
Examples
Embodiment Construction
[0020] Combine below figure 1 The image labeling method based on characteristic scene of the present invention is described in more detail:
[0021] In step 1, an image segmentation algorithm is used to segment the labeled image used for learning to obtain a visual description of the image area.
[0022] Step 2, perform automatic semantic scene clustering on the labeled images used for learning. The specific method is as follows.
[0023] In the set of labeled images used for learning, high-density regions are selected as semantic scene clustering centers. In the present invention, the method of Shared Nearest Neighbor (SNN) is used to first construct a sample similarity matrix, and the nearest neighbor of each sample can adopt the intersection of its visual neighbor and semantically marked neighbor. Then, the sparse processing of k nearest neighbors is performed, and the nearest neighbor graph is constructed from this. By calculating the link strength of all sample points...
PUM
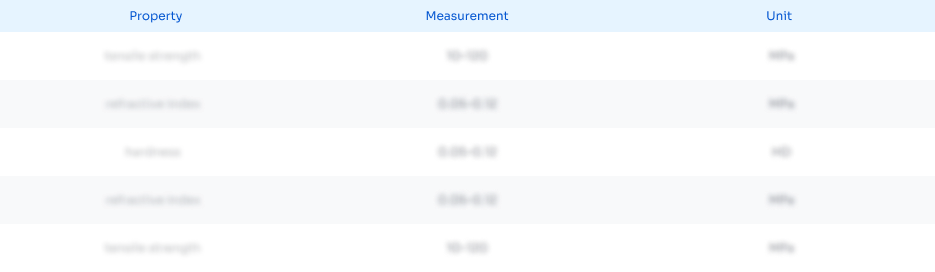
Abstract
Description
Claims
Application Information
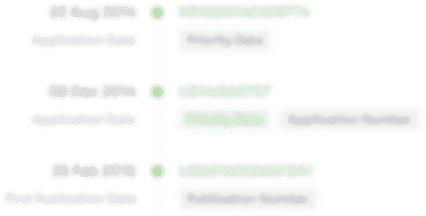
- R&D Engineer
- R&D Manager
- IP Professional
- Industry Leading Data Capabilities
- Powerful AI technology
- Patent DNA Extraction
Browse by: Latest US Patents, China's latest patents, Technical Efficacy Thesaurus, Application Domain, Technology Topic, Popular Technical Reports.
© 2024 PatSnap. All rights reserved.Legal|Privacy policy|Modern Slavery Act Transparency Statement|Sitemap|About US| Contact US: help@patsnap.com