Method for segmenting images by utilizing sparse representation and dictionary learning
An image segmentation and sparse representation technology, applied in the field of image processing, can solve the problems of unstable results, inapplicability, accuracy dependence, etc., to achieve accurate segmentation results, wide application of algorithms, and stable image segmentation effects.
- Summary
- Abstract
- Description
- Claims
- Application Information
AI Technical Summary
Problems solved by technology
Method used
Image
Examples
Embodiment Construction
[0023] Refer to attached figure 1 , concrete steps of the present invention include:
[0024] Step 1. Input the image to be segmented with a size of N×N, and extract the feature matrix M of the image to be segmented
[0025] The texture features extracted from the image to be segmented in the present invention include grayscale co-occurrence features and wavelet features:
[0026] 1a) Use the gray level co-occurrence matrix to extract features from the image to be segmented
[0027] Generate a gray level co-occurrence matrix p for the image to be segmented ij (s, θ), where s is the pixel point x i and x j The distance between, the value of θ is 4 discrete directions: 0°, 45°, 90°, 135°, and three statistics are taken in each direction: second-order moment of angle, homogeneous area, contrast, Each statistic is calculated according to the following formula:
[0028] Angular second moment: f 1 = Σ i ...
PUM
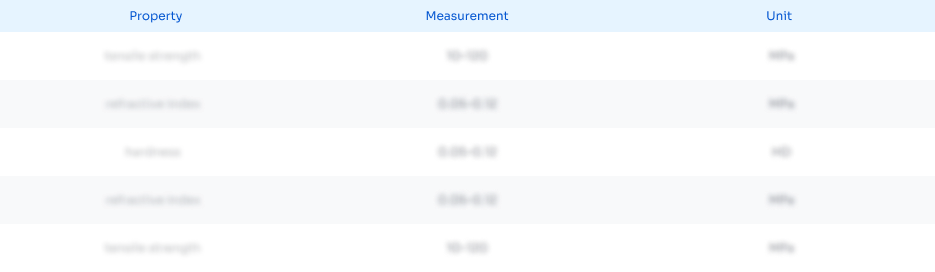
Abstract
Description
Claims
Application Information
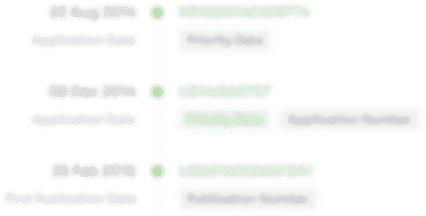
- R&D
- Intellectual Property
- Life Sciences
- Materials
- Tech Scout
- Unparalleled Data Quality
- Higher Quality Content
- 60% Fewer Hallucinations
Browse by: Latest US Patents, China's latest patents, Technical Efficacy Thesaurus, Application Domain, Technology Topic, Popular Technical Reports.
© 2025 PatSnap. All rights reserved.Legal|Privacy policy|Modern Slavery Act Transparency Statement|Sitemap|About US| Contact US: help@patsnap.com