Image annotation method based on sparse group structure
A technology of image labeling and grouping, which is applied to instruments, computing, electrical and digital data processing, etc., can solve the problems that affect the labeling results, do not consider the relevance of labeling words, etc., and achieve the effect of accurate labeling results.
- Summary
- Abstract
- Description
- Claims
- Application Information
AI Technical Summary
Problems solved by technology
Method used
Image
Examples
Embodiment
[0051] The image annotation method based on the sparse group structure includes the following steps:
[0052] 1) Feature extraction is performed on image data, including global features and local features. Full-play features include color histogram, color moment, color correlation map, and wavelet transform, and local features include SIFT and shape context;
[0053] 2) Each image is represented by a combination of extracted heterogeneous feature vectors. That is, an image i is denoted as (x i ,y i )∈R p × {0, 1} C , where x i =(x i1 ,...,x ip ) T ∈R p Represents the feature vector of the image, p represents the feature dimension, y i =(y i1 ,...,y iC ) T ∈ {0, 1} C is the corresponding labeled word vector, C represents the total number of labeled words in the data set, y ij = 1 means that the i-th image has the j-th label, otherwise, y ij =0. Assuming that the image has G class feature representation, d g Represents the dimension of the g-th class feature, g∈...
PUM
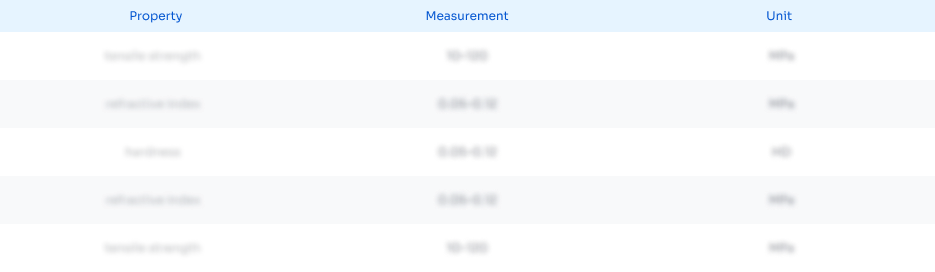
Abstract
Description
Claims
Application Information
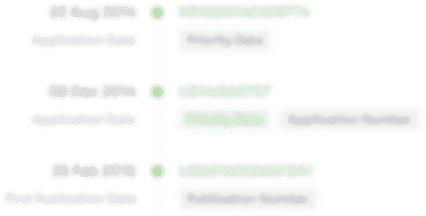
- R&D Engineer
- R&D Manager
- IP Professional
- Industry Leading Data Capabilities
- Powerful AI technology
- Patent DNA Extraction
Browse by: Latest US Patents, China's latest patents, Technical Efficacy Thesaurus, Application Domain, Technology Topic.
© 2024 PatSnap. All rights reserved.Legal|Privacy policy|Modern Slavery Act Transparency Statement|Sitemap