Image super-resolution reconstruction method based on dictionary learning and structure similarity
A technology of super-resolution reconstruction and similar structure, applied in the field of image processing, can solve the problems of not being able to keep high-frequency details of high-resolution images well, low efficiency, high computational complexity, etc., to achieve rich content, accurate sparse coefficients, Clear high-resolution images
- Summary
- Abstract
- Description
- Claims
- Application Information
AI Technical Summary
Problems solved by technology
Method used
Image
Examples
Embodiment Construction
[0037] Attached below figure 1 The steps of the present invention are further described in detail:
[0038] Step 1. Collect training sample pairs M=[M from the sample database h ; l ]=[m 1 ,...,m num ], where M h Denotes a high-resolution sample block, M l Indicates the corresponding low-resolution sample block, m p Indicates the pth column of M, 1≤p≤num, and num indicates the number of sample pairs. In the simulation experiment, the number of training sample pairs collected is num=100000.
[0039] Step 2. Use the method of SSIM and K-SVD with similar structures and the training sample pair M in step (1) to obtain the dictionary D 1 .
[0040] (2a) initial dictionary D;
[0041] (2b) Use structurally similar SSIM to solve the training sample pair M column vectors m p Sparse representation coefficient α under dictionary D p , get the sparse coefficient α=[α 1 ,...,α num ], solve the training sample pair M each column vector m p Sparse representation coefficient α ...
PUM
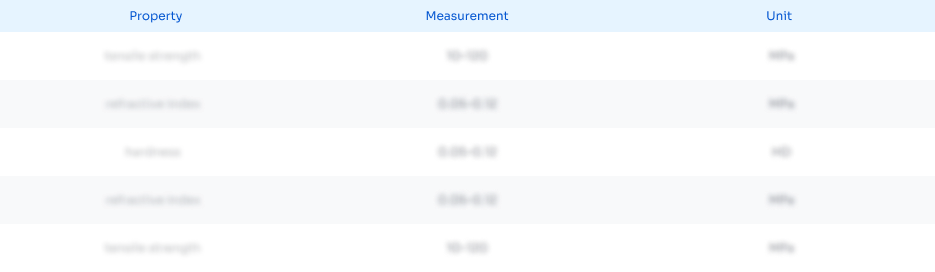
Abstract
Description
Claims
Application Information
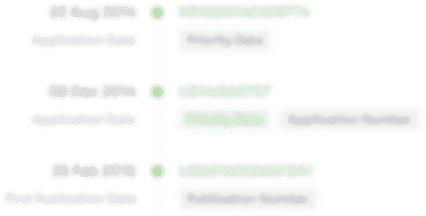
- R&D Engineer
- R&D Manager
- IP Professional
- Industry Leading Data Capabilities
- Powerful AI technology
- Patent DNA Extraction
Browse by: Latest US Patents, China's latest patents, Technical Efficacy Thesaurus, Application Domain, Technology Topic, Popular Technical Reports.
© 2024 PatSnap. All rights reserved.Legal|Privacy policy|Modern Slavery Act Transparency Statement|Sitemap|About US| Contact US: help@patsnap.com