Fuzzy clustering image segmenting method
A technology of image segmentation and fuzzy clustering, applied in image analysis, image data processing, instrumentation, etc., can solve problems such as unknown position and characteristics of class centers, sensitivity to noise, high computational overhead, etc.
- Summary
- Abstract
- Description
- Claims
- Application Information
AI Technical Summary
Problems solved by technology
Method used
Image
Examples
Embodiment
[0050] The image segmentation method of the present invention is used to segment the early diabetic retinopathy (hard exudation and cotton wool spots) in the noise-free color fundus image with a resolution of 640×480 pixels.
[0051] It can be seen from Fig. 2(a), Fig. 2(b), Fig. 2(c) that the two algorithms are effective for early diabetic retinopathy (hard exudates and cotton wool spots) in color fundus images without noise pollution. The segmentation results are almost the same. As can be seen from Table 1 (table 1 is the segmentation result statistics of FCM and the present invention to early stage diabetic retinopathy (hard exudation and velvet spots) in 55 color fundus images without noise pollution), the number of iterations of the present invention and Clustering time is significantly less than FCM. Therefore, it can be concluded that the present invention can increase the segmentation speed of the traditional FCM algorithm by about 2.3 times without affecting the seg...
PUM
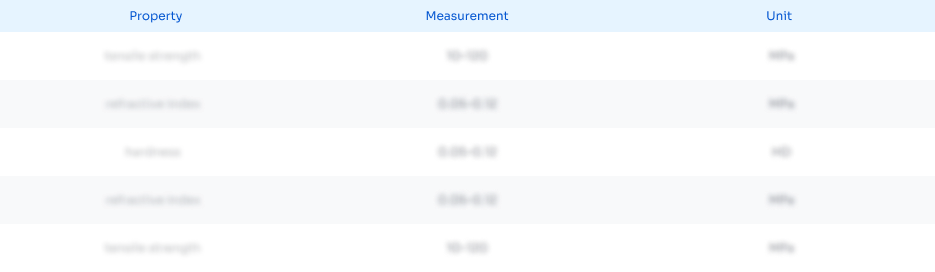
Abstract
Description
Claims
Application Information
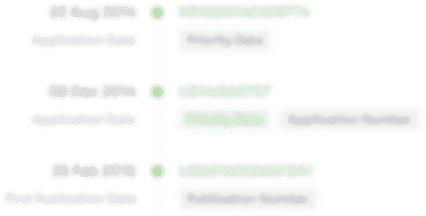
- R&D
- Intellectual Property
- Life Sciences
- Materials
- Tech Scout
- Unparalleled Data Quality
- Higher Quality Content
- 60% Fewer Hallucinations
Browse by: Latest US Patents, China's latest patents, Technical Efficacy Thesaurus, Application Domain, Technology Topic, Popular Technical Reports.
© 2025 PatSnap. All rights reserved.Legal|Privacy policy|Modern Slavery Act Transparency Statement|Sitemap|About US| Contact US: help@patsnap.com