Continuous voice recognition method based on deep long and short term memory recurrent neural network
A technology of cyclic neural network and long-term short-term memory, which is applied in speech recognition, speech analysis, instruments, etc., can solve the problem of interfering continuous speech recognition system performance, acoustic model noise resistance and robustness need to be improved, deep neural network method parameters large scale issues
- Summary
- Abstract
- Description
- Claims
- Application Information
AI Technical Summary
Problems solved by technology
Method used
Image
Examples
Embodiment Construction
[0034] The implementation of the present invention will be described in detail below in conjunction with the drawings and examples.
[0035] The present invention proposes a method and device for a robust deep long short-term memory neural network acoustic model, especially for continuous speech recognition scenarios. These methods and devices are not limited to continuous speech recognition, and may be any methods and devices related to speech recognition.
[0036] Step 1. Establish two deep long-term short-term memory recurrent neural network modules with the same structure including multiple long-term short-term memory layers and linear recurrent projection layers, and send the original pure speech signal and noisy signal as input to the two modules.
[0037] figure 1 It is a flow chart of the depth long short-term memory recurrent neural network module of the present invention, including the following:
[0038] Input 101 is speech signal x=[x 1 ,...,x T ] (T is the ti...
PUM
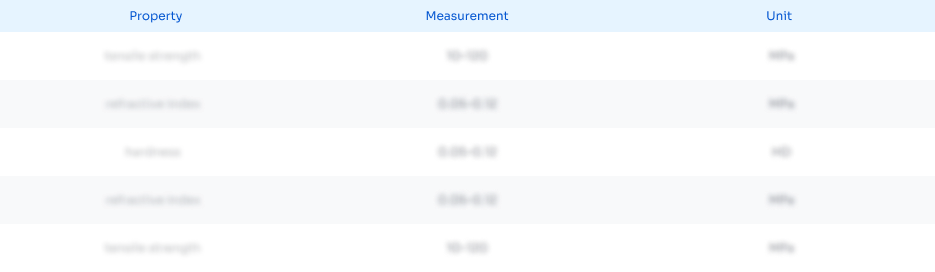
Abstract
Description
Claims
Application Information
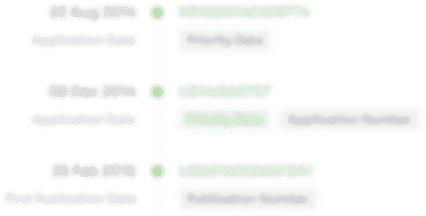
- R&D Engineer
- R&D Manager
- IP Professional
- Industry Leading Data Capabilities
- Powerful AI technology
- Patent DNA Extraction
Browse by: Latest US Patents, China's latest patents, Technical Efficacy Thesaurus, Application Domain, Technology Topic.
© 2024 PatSnap. All rights reserved.Legal|Privacy policy|Modern Slavery Act Transparency Statement|Sitemap