Task scheduling method of self-learning feedback under Hadoop multi-job environment
A task scheduling and self-learning technology, applied in multi-programming devices, resource allocation, etc., can solve problems such as self-evident limitations, different actual weights, and no reference value, so as to improve accuracy and hit hits. rate, and the effect of promoting optimal utilization
- Summary
- Abstract
- Description
- Claims
- Application Information
AI Technical Summary
Problems solved by technology
Method used
Image
Examples
Embodiment Construction
[0015] The present invention will be further described below in conjunction with the accompanying drawings and embodiments.
[0016] Please refer to figure 1 After each working node of Hadoop of the present invention passes through the analysis of the job submission stage, each job is independently studied, and the ratio of the execution time of each sub-stage is calculated for the completed Map task or Reduce task, and converted into a stage weight, Use the geometric mean algorithm to process the stage weights of the above completed tasks, and obtain the reference stage weights applicable to each sub-stage of the job in the current Hadoop cluster environment. In the task feedback phase, the reference phase weights obtained from the self-learning phase are used to set phase weights for the remaining tasks of the job that conform to the task execution characteristics and the current resource environment. By using the weight of the stage combined with the progress of the sub-st...
PUM
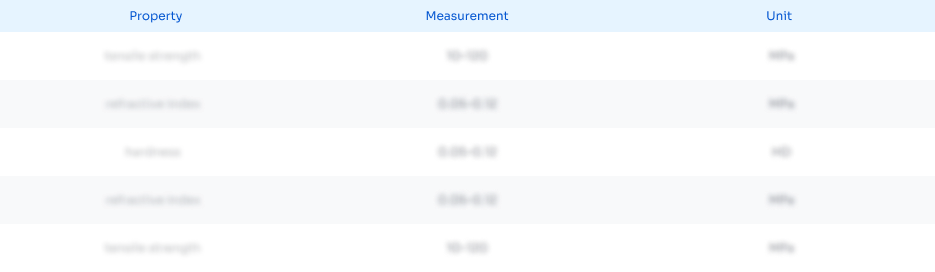
Abstract
Description
Claims
Application Information
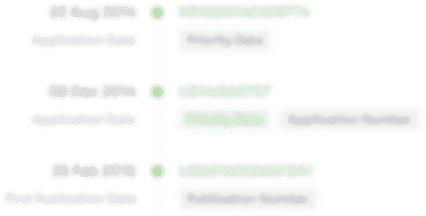
- R&D Engineer
- R&D Manager
- IP Professional
- Industry Leading Data Capabilities
- Powerful AI technology
- Patent DNA Extraction
Browse by: Latest US Patents, China's latest patents, Technical Efficacy Thesaurus, Application Domain, Technology Topic, Popular Technical Reports.
© 2024 PatSnap. All rights reserved.Legal|Privacy policy|Modern Slavery Act Transparency Statement|Sitemap|About US| Contact US: help@patsnap.com