Adaboost classifier on-line learning method and Adaboost classifier on-line learning system
A learning method and classifier technology, applied in the field of classifiers, can solve the problems of low detection rate, high false positive rate, low false positive rate, etc., and achieve the effect of improving the detection rate, reducing the false positive rate, and improving the generalization performance.
- Summary
- Abstract
- Description
- Claims
- Application Information
AI Technical Summary
Problems solved by technology
Method used
Image
Examples
Example Embodiment
[0030] The technical solutions of the Adaboost classifier online learning method and system will be described in detail below in conjunction with specific embodiments and drawings to make it clearer.
[0031] Such as figure 1 Shown is a flowchart of an Adaboost classifier online learning method in an embodiment. The online learning method of the Adaboost classifier includes:
[0032] Step S102, using the strong classifier obtained by offline training to perform target detection, and obtain the target detection result.
[0033] In step S104, the background model is used for target detection to obtain a moving target.
[0034] Specifically, the background model is a Gaussian mixture model, a background difference method model, or a frame average background model.
[0035] First, when the background model is a Gaussian mixture model, such as figure 2 As shown, the background model is used for target detection, and the steps to obtain a moving target include:
[0036] Step S202, if the cur...
PUM
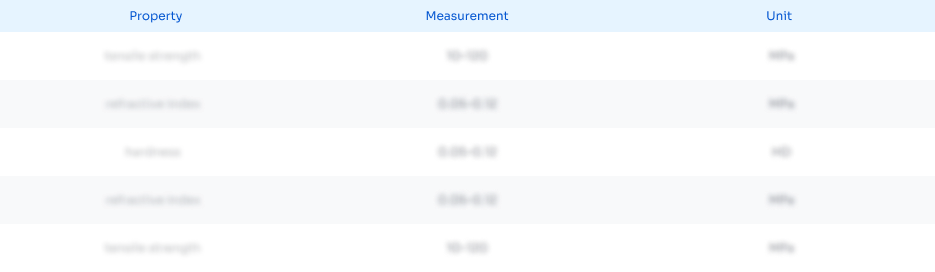
Abstract
Description
Claims
Application Information
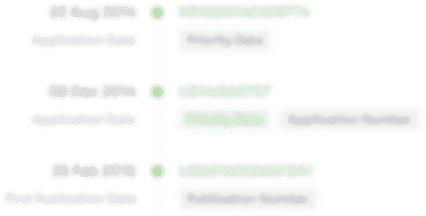
- R&D Engineer
- R&D Manager
- IP Professional
- Industry Leading Data Capabilities
- Powerful AI technology
- Patent DNA Extraction
Browse by: Latest US Patents, China's latest patents, Technical Efficacy Thesaurus, Application Domain, Technology Topic.
© 2024 PatSnap. All rights reserved.Legal|Privacy policy|Modern Slavery Act Transparency Statement|Sitemap