Feature selection method and device
A feature selection method and feature orientation technology, applied in the field of medical diagnosis, can solve the problems of poor promotion ability, high calculation and payment, and low learning efficiency.
- Summary
- Abstract
- Description
- Claims
- Application Information
AI Technical Summary
Problems solved by technology
Method used
Image
Examples
Embodiment 1
[0055] figure 1 This is a flowchart of a feature selection method provided in Embodiment 1 of the present application.
[0056] like figure 1 As shown, the method includes:
[0057] Step A: In response to the received training sample set including a plurality of training samples with the same characteristics, according to the category of the training samples in the training sample set, the training sample set is divided into a first training sample set and a second training sample set, and according to the training sample set The features of the training samples in the sample set are used to generate a first feature index set corresponding to the first training sample set, and a second feature index set corresponding to the second training sample set.
[0058] In the embodiment of the present application, firstly, in response to the received training sample set, the training sample set may be input by the user through import, or may be input by manual input, and the training...
Embodiment 2
[0107] figure 2 This is a schematic structural diagram of a feature selection apparatus provided in Embodiment 2 of the present application.
[0108] like figure 2 As shown, the device includes:
[0109] Response unit 1, for performing step A in response to the received training sample set including a plurality of training samples with the same characteristics, and dividing the training sample set into a first training sample set and a second training sample set according to the categories of the training samples in the training sample set A sample set is generated, and a first feature index set corresponding to the first training sample set and a second feature index set corresponding to the second training sample set are generated according to the features of the training samples in the training sample set.
[0110] The statistics unit 2 is connected with the response unit 1, and is used for performing step B of counting the sum of the number of features corresponding to...
Embodiment 3
[0132] The embodiment of the present application mainly uses the diagnosis module to verify the result of the feature selection of the present application, and further illustrates the learning efficiency of the feature selection result of the embodiment of the present application.
[0133] In the embodiment of the present application, after the feature selection is completed and the feature index set F is obtained, the feature index set F includes r' elements, and because the training sample set The training sample set after feature selection is determined according to the elements in the feature index set F. Therefore, the training sample set determined according to the feature index set F is: { x ‾ t , y t } i = 1 ...
PUM
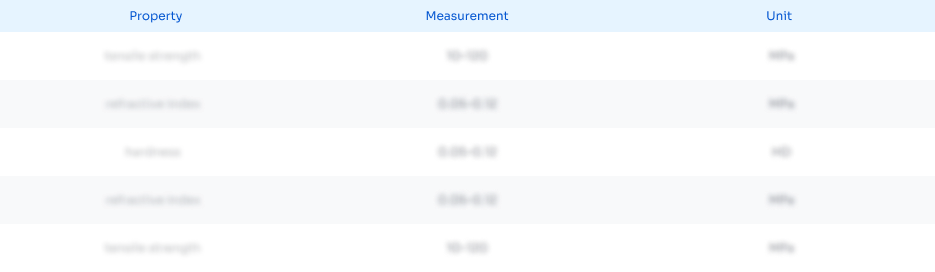
Abstract
Description
Claims
Application Information
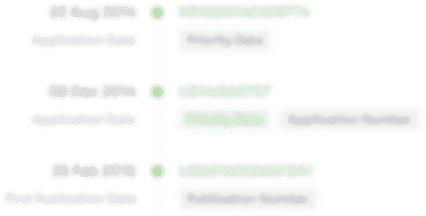
- R&D Engineer
- R&D Manager
- IP Professional
- Industry Leading Data Capabilities
- Powerful AI technology
- Patent DNA Extraction
Browse by: Latest US Patents, China's latest patents, Technical Efficacy Thesaurus, Application Domain, Technology Topic, Popular Technical Reports.
© 2024 PatSnap. All rights reserved.Legal|Privacy policy|Modern Slavery Act Transparency Statement|Sitemap|About US| Contact US: help@patsnap.com