Hyperspectral remote sensing image classifying method based on hierarchy ensemble learning
A technology of hyperspectral remote sensing and classification method, which is applied in the fields of instruments, character and pattern recognition, computer parts, etc. The effect of classification accuracy
- Summary
- Abstract
- Description
- Claims
- Application Information
AI Technical Summary
Problems solved by technology
Method used
Image
Examples
specific Embodiment approach 1
[0032] Specific implementation mode one: the following combination figure 1 Describe this embodiment, the hyperspectral remote sensing image classification method based on hierarchical integrated learning described in this embodiment, it comprises the following steps:
[0033] Step 1: Read the hyperspectral raw data, preprocess it, obtain supervised data, then determine the labeled samples according to the supervised data, and select training samples and test samples from the labeled samples;
[0034] Step 2: Perform random band selection for all spectral bands of the hyperspectral raw data, and then extract a pixel vector combination from each marked sample, the number of bands contained in each pixel vector in the pixel vector combination is the same as that of the random The number of bands corresponds one by one to form a spectral set; repeat the random band selection process and form a corresponding spectral set to form multiple spectral sets with differences;
[0035] S...
specific Embodiment approach 2
[0039] Specific implementation mode two: this implementation mode further explains implementation mode one, the specific method of determining the marked samples in step one, and selecting training samples and test samples from the marked samples is as follows:
[0040] Read the hyperspectral raw data stored in the form of a three-dimensional matrix, the three-dimensional matrix form includes two-dimensional spatial position information and one-dimensional spectral information; The object categories in the marker map are respectively marked in the form of integer values, and the supervision data in the form of a two-dimensional matrix is obtained. composition;
[0041] From the hyperspectral raw data and supervisory data, determine the number of feature categories C in the real feature marker map, the number of pixels in the two-dimensional spatial position information M rows × N columns, and the number of effective bands in the one-dimensional spectral information B;
[004...
specific Embodiment approach 3
[0047] Specific implementation mode three: the following combination figure 1 Describe this embodiment, this embodiment will further explain Embodiment 2, and the specific method for forming multiple spectral sets with differences in Step 2 is as follows:
[0048] Draw a set of b random numbers between 1 and B {i 1 ,i 2 ,...,i b}, where 1≤i k ≤B, k=1,2,...,b), and then from each labeled sample, the i-th k The vector elements are extracted to form a pixel vector combination containing b elements respectively, the number of the pixel vector combination is the same as the number of marked samples, and all the pixel vector combinations containing b elements are used as a spectral set;
[0049] The process of forming a spectrum set is repeated multiple times, and b random numbers are selected in the repeated process, and each combination of random numbers is different, so as to obtain multiple spectrum sets with differences.
[0050] The hyperspectral raw data contains dozens ...
PUM
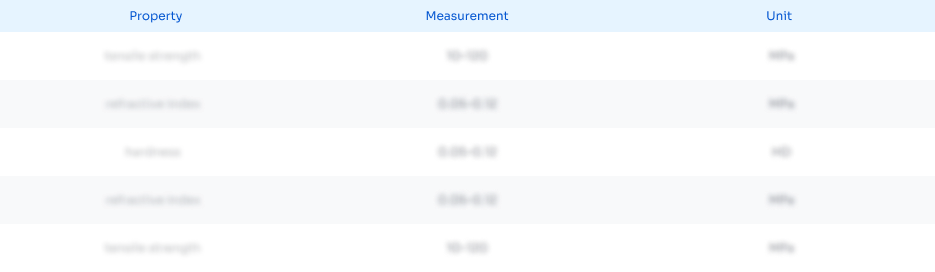
Abstract
Description
Claims
Application Information
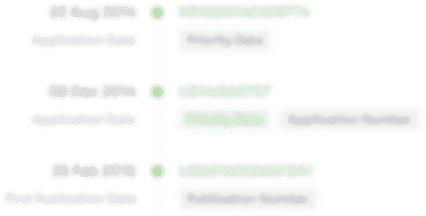
- R&D Engineer
- R&D Manager
- IP Professional
- Industry Leading Data Capabilities
- Powerful AI technology
- Patent DNA Extraction
Browse by: Latest US Patents, China's latest patents, Technical Efficacy Thesaurus, Application Domain, Technology Topic, Popular Technical Reports.
© 2024 PatSnap. All rights reserved.Legal|Privacy policy|Modern Slavery Act Transparency Statement|Sitemap|About US| Contact US: help@patsnap.com