Scene classification method based on Gist characteristics and extreme learning machine
An extreme learning machine and scene classification technology, applied in the field of extreme learning machine classification, can solve problems such as few scene classification methods and are not so flexible, and achieve the effect of easy multi-classification problems, simple parameter setting, and fast operation speed
- Summary
- Abstract
- Description
- Claims
- Application Information
AI Technical Summary
Problems solved by technology
Method used
Image
Examples
Example Embodiment
[0041] For the test of the present invention, MIT’s OT image library is used. The image scene library is divided into eight types of scenes: 360 for beaches, 328 for forests, 374 for mountains, 410 for outdoors, 260 for highways, 308 for urban areas, and streets. 292, 356 high-rise buildings, a total of 2688.
[0042] figure 1 It is the classification display result of a single test picture of the two types of classification process of the present invention, figure 1 It contains natural scenes and man-made scenes. The text on the header of the picture is described as a classified scene. The first 3 of the 4 pictures are divided correctly, and the lower right corner is divided incorrectly. The two-class classification process refers to merging the eight types of scene images into two types of natural scenes and artificial scenes. Natural scenes include beaches, forests, mountains, and outdoors; artificial scenes include highways, urban areas, streets, and tall buildings.
[0043] ...
PUM
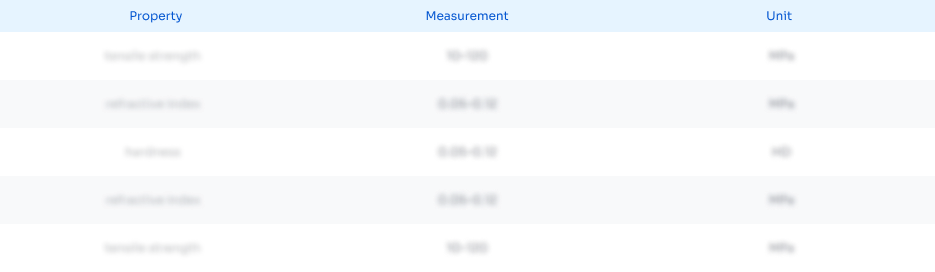
Abstract
Description
Claims
Application Information
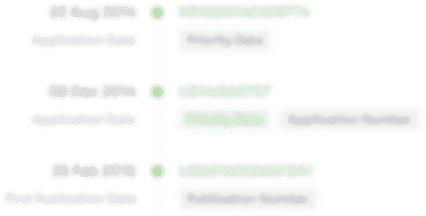
- R&D Engineer
- R&D Manager
- IP Professional
- Industry Leading Data Capabilities
- Powerful AI technology
- Patent DNA Extraction
Browse by: Latest US Patents, China's latest patents, Technical Efficacy Thesaurus, Application Domain, Technology Topic.
© 2024 PatSnap. All rights reserved.Legal|Privacy policy|Modern Slavery Act Transparency Statement|Sitemap