Polarized SAR Classification Method Based on NSCT and Discriminative Dictionary Learning
A technology of dictionary learning and classification methods, applied in the fields of image processing and target recognition, can solve the problems affecting data classification effect, classification speed, and classification effect, and achieve the effect of facilitating understanding, improving classification speed, and improving classification accuracy.
- Summary
- Abstract
- Description
- Claims
- Application Information
AI Technical Summary
Problems solved by technology
Method used
Image
Examples
Embodiment Construction
[0022] The technical solutions and effects of the present invention will be clearly and completely described below in conjunction with the accompanying drawings.
[0023] refer to figure 1 , the implementation steps of the present invention are as follows:
[0024] Step 1: Input the polarimetric SAR image to be classified, obtain the coherence matrix of each pixel, and perform Lee filtering on the coherence matrix to obtain the denoised coherence matrix.
[0025] Step 2, perform Cloude decomposition on the denoised coherence matrix to obtain 3 non-negative eigenvalues and 3 eigenvectors.
[0026] Decompose the denoised coherence matrix to obtain classification features. The decomposition methods include Freeman decomposition, Cloude decomposition, four-component decomposition, etc.;
[0027] The present invention uses Cloude decomposition to decompose each pixel of the polarimetric SAR image to obtain three non-negative eigenvalues λ 1 ,λ 2 ,λ 3 and 3 eigenvectors v ...
PUM
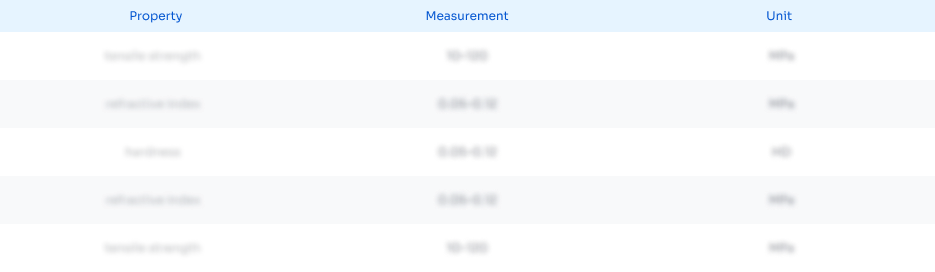
Abstract
Description
Claims
Application Information
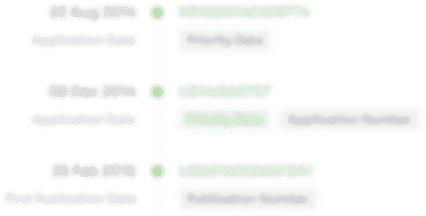
- R&D Engineer
- R&D Manager
- IP Professional
- Industry Leading Data Capabilities
- Powerful AI technology
- Patent DNA Extraction
Browse by: Latest US Patents, China's latest patents, Technical Efficacy Thesaurus, Application Domain, Technology Topic, Popular Technical Reports.
© 2024 PatSnap. All rights reserved.Legal|Privacy policy|Modern Slavery Act Transparency Statement|Sitemap|About US| Contact US: help@patsnap.com