Ordinary object recognizing method based on 2D and 3D SIFT feature fusion
A feature fusion, object recognition technology, applied in character and pattern recognition, instruments, computer parts and other directions
- Summary
- Abstract
- Description
- Claims
- Application Information
AI Technical Summary
Problems solved by technology
Method used
Image
Examples
Embodiment 1
[0077] Embodiment 1 Recognition Algorithm Framework
[0078] A general object recognition method based on fusion of 2D and 3D SIFT features mainly includes the following steps:
[0079] 1) Feature extraction and representation:
[0080] Feature extraction and representation are the basis of object recognition. How to extract stable and effective features is the focus and difficulty in feature extraction research. Only by selecting good features can the best recognition results be obtained under the condition of limited training samples. Generally, there are a large number of objects, and it is impossible to build a model library for each object. At the same time, the shape and color of each object of each class are also very different, so the extracted object features must meet the following conditions: 1) Make the difference between the classes the largest, that is, the most It can represent the characteristics of each type of object that are different from other types of ob...
Embodiment 2
[0148] Embodiment 2 algorithm flow
[0149] like figure 2 Shown is a schematic flow chart of a general object recognition algorithm based on 2D and 3D SIFT feature fusion. The general object recognition process proposed by the present invention mainly includes two stages of offline training and online recognition. The following is a detailed description of the training link and the recognition link in the flow chart.
[0150] 1. Training algorithm process:
[0151] 1.1 Offline training phase:
[0152] 1.1.1 After the offline training starts, the image p corresponding to the i-th class object in the object image database i The point cloud pc corresponding to the i-th object in the object point cloud library i , i=1, 2, K n, n represents the number of training sample categories, first extract the 2D and 3D SIFT features corresponding to n types of training samples, recorded as F_R={f i _R,i=1,2,K n},R∈(2D,3D), where, f i _2D is m i *128 feature vector sets, f i _3D is m...
Embodiment 3
[0160] Embodiment 3 experimental result
[0161] The point cloud model and RGB image used in the experiment of the present invention come from K.lai et al. (RGB-D dataset.http: / / rgbd-dataset.cs.washington.edu / dataset.html, 2011-03-05. For K.Lai, L.-F.~Bo, X.-F~Ren, D.~Fox, A Large-Scale Hierarchical Multi-View RGB-D Object Dataset, Proc.of IEEE Int.Conf.on Robotics and Autom., pp: 1817--1824, Shanghai, China, 2011.) established a large point cloud database, which contains 51 categories of point cloud models and RGB images of 300 objects, and each object point cloud and image contains 3 perspectives. Experimental method: randomly select one object in each category as a test sample, and the remaining objects as a training sample, select 100 training samples for each category, and 60 test samples, all randomly selected from the database. In order to evaluate the performance of the algorithm proposed in this paper, a number of experiments were carried out in this part, and the c...
PUM
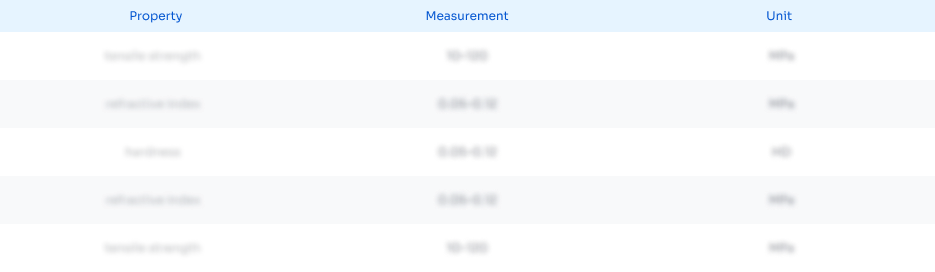
Abstract
Description
Claims
Application Information
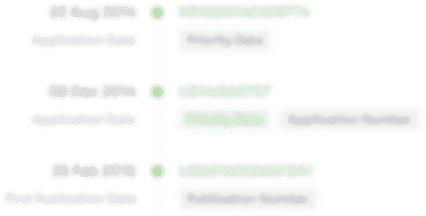
- R&D Engineer
- R&D Manager
- IP Professional
- Industry Leading Data Capabilities
- Powerful AI technology
- Patent DNA Extraction
Browse by: Latest US Patents, China's latest patents, Technical Efficacy Thesaurus, Application Domain, Technology Topic, Popular Technical Reports.
© 2024 PatSnap. All rights reserved.Legal|Privacy policy|Modern Slavery Act Transparency Statement|Sitemap|About US| Contact US: help@patsnap.com