Reasoning algorithm based on importance sampling and neural circuit
An importance sampling and circuit technology, applied in the field of human brain science, can solve the problems of small scale, not in line with the efficient working principle of the human brain, and the circuit has no universality, and achieves the effect of good universality.
- Summary
- Abstract
- Description
- Claims
- Application Information
AI Technical Summary
Problems solved by technology
Method used
Image
Examples
Embodiment 1
[0047] Such as image 3 The shown Bayesian network first decomposes the Bayesian network into a basic tree-shaped Bayesian network. This basic network consists of a top-level parent node and several child nodes. The decomposed results are as follows Figure 4 shown.
[0048]Further, probabilistic reasoning is carried out on the lowest layer structure, and the reasoning result is:
[0049] Σ C 1 , C 2 P ( B 1 | C 1 ...
Embodiment 2
[0068] This embodiment is a neural circuit corresponding to the inference algorithm based on importance sampling described in Embodiment 1. combine Image 6 As shown, in this embodiment, the neural circuit includes an input layer, an intermediate layer and a decision layer. Among them, the input layer codes the probability of external stimuli (observations), the middle layer calculates the probability linearly, and the decision-making layer determines the result of reasoning.
[0069] More specifically, each Poisson neuron in the input layer codes the external stimulus (observation) information probabilistically, and the output response of the Poisson neuron is:
[0070] r = {r 1 ,r 2 ,...,r N},
[0071] The probability distribution for the response r is:
[0072] P ( r | S ) = Π i e ...
PUM
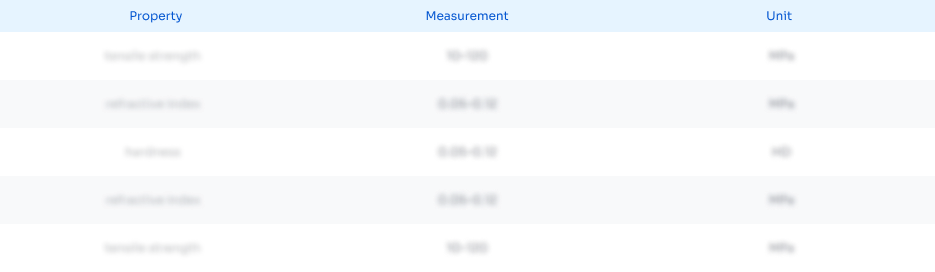
Abstract
Description
Claims
Application Information
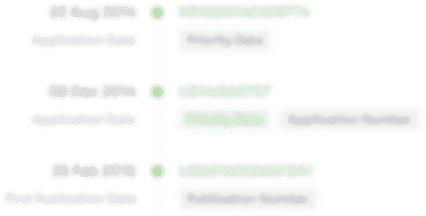
- R&D
- Intellectual Property
- Life Sciences
- Materials
- Tech Scout
- Unparalleled Data Quality
- Higher Quality Content
- 60% Fewer Hallucinations
Browse by: Latest US Patents, China's latest patents, Technical Efficacy Thesaurus, Application Domain, Technology Topic, Popular Technical Reports.
© 2025 PatSnap. All rights reserved.Legal|Privacy policy|Modern Slavery Act Transparency Statement|Sitemap|About US| Contact US: help@patsnap.com