Kernel function based rare category detection method fusing active learning and nonparametric semi-supervised clustering
A semi-supervised clustering, active learning technology, applied in character and pattern recognition, instruments, computer parts, etc., can solve the problem of not finding rare categories
- Summary
- Abstract
- Description
- Claims
- Application Information
AI Technical Summary
Problems solved by technology
Method used
Image
Examples
Embodiment
[0063] Embodiment: A rare category detection method based on kernel function fusion active learning and non-semi-supervised clustering. The method has three stages: semi-supervised clustering hierarchical construction, active learning based on multiple standards, and iterative control process.
[0064] Among them, the semi-supervised clustering hierarchical construction stage includes the kernel function-based distance metric learning sub-stage and the non-parametric clustering hierarchical construction sub-stage.
[0065] 1) Distance metric learning based on kernel function, the process is as follows figure 1 shown.
[0066] Step 1, calculate the kernel matrix K of the dataset X in the kernel space based on the selected kernel function; if it is a linear mapping, directly output the matrix, otherwise perform steps 2-9.
[0067] Using the mapping function φ(x), the data set X=(x 1 ,x 2 ,...,x n ) from the original Euclidean space to the inner product space (kernelspace). ...
PUM
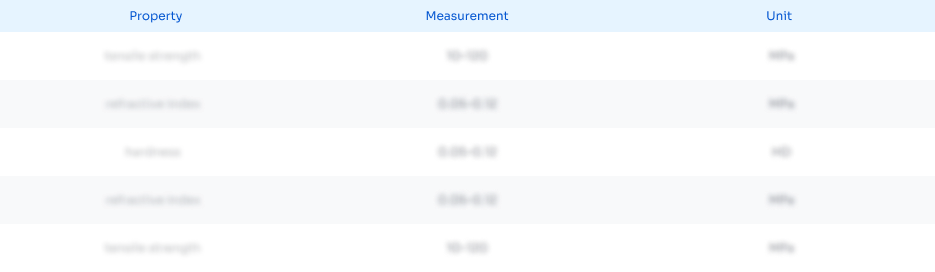
Abstract
Description
Claims
Application Information
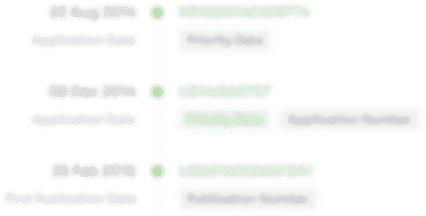
- R&D Engineer
- R&D Manager
- IP Professional
- Industry Leading Data Capabilities
- Powerful AI technology
- Patent DNA Extraction
Browse by: Latest US Patents, China's latest patents, Technical Efficacy Thesaurus, Application Domain, Technology Topic, Popular Technical Reports.
© 2024 PatSnap. All rights reserved.Legal|Privacy policy|Modern Slavery Act Transparency Statement|Sitemap|About US| Contact US: help@patsnap.com