Characteristic selecting method and characteristic selecting device based on artificial neural network
A technology of artificial neural network and feature selection method, applied in the field of feature selection based on artificial neural network, can solve the problem of low efficiency of feature selection
- Summary
- Abstract
- Description
- Claims
- Application Information
AI Technical Summary
Problems solved by technology
Method used
Image
Examples
Embodiment 1
[0106] Figure 1a A schematic flowchart of a feature selection method based on an artificial neural network according to an embodiment of the present invention is shown. Such as Figure 1a As shown, the feature selection method based on artificial neural network may mainly include:
[0107] Step 101: Construct an artificial neural network with an input layer, an intermediate layer, and an output layer according to the K features to be selected and O output targets, wherein the input layer includes K nodes and each node corresponds to a feature, the The output layer includes O nodes and each node corresponds to an output target.
[0108] Step 102: Use the training set to train the artificial neural network to determine the connection weights from each layer to the next layer in the artificial neural network, wherein the optimization function used during training includes the sparsity of the input layer The item of constraint, so that the connection weight of the input layer to the ...
Embodiment 2
[0129] figure 2 A schematic flowchart of a feature selection method based on an artificial neural network according to another embodiment of the present invention is shown. figure 2 Win the mark and Figure 1a The same steps have the same functions. For brevity, detailed descriptions of these steps are omitted.
[0130] Such as figure 2 As shown, the difference from the foregoing embodiment is that after step 102 uses the training set to train the artificial neural network to determine the connection weights from each layer to the next layer in the artificial neural network, the artificial neural network is based on The feature selection method may further include: adding a test set including at least one test sample; according to the training set and the test set, using the trained artificial neural network to calculate the K features for the O output The impact of the target can specifically include:
[0131] Step 201: Calculate the first loss function represented by Formula 3...
Embodiment 3
[0152] Figure 3a A schematic flowchart of a feature selection method based on a deep learning network according to another embodiment of the present invention is shown. Figure 3a Win the mark and Figure 1a , figure 2 The same steps have the same functions. For brevity, detailed descriptions of these steps are omitted.
[0153] Such as Figure 3b As shown, a deep learning (Deep Learning with Feature Selection) network with feature selection can be constructed. The deep learning (Deep Learning) network is an artificial neural network with an intermediate layer (or hidden layer) greater than one. The deep learning network generally first initializes the parameters of each layer through unsupervised learning; then through supervised learning to fine-tune the overall parameters and optimize the parameters of all layers at the same time. In the embodiment of the present invention, feature selection (FeatureSelection) can be added to the input layer during unsupervised learning to in...
PUM
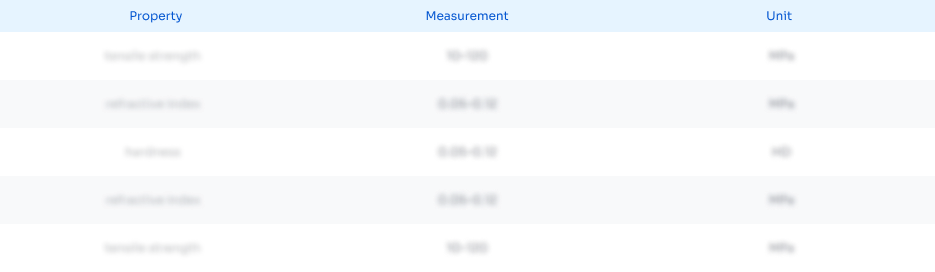
Abstract
Description
Claims
Application Information
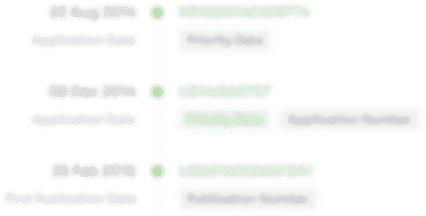
- R&D Engineer
- R&D Manager
- IP Professional
- Industry Leading Data Capabilities
- Powerful AI technology
- Patent DNA Extraction
Browse by: Latest US Patents, China's latest patents, Technical Efficacy Thesaurus, Application Domain, Technology Topic, Popular Technical Reports.
© 2024 PatSnap. All rights reserved.Legal|Privacy policy|Modern Slavery Act Transparency Statement|Sitemap|About US| Contact US: help@patsnap.com