Statistical compressed sensing image reconstruction method based on layered Gauss mixing model
A Gaussian mixture model and compressed sensing technology, applied in the field of image processing, can solve the problems of non-Gaussian and low quality of reconstructed images, and achieve the effect of less measurement, simple iterative form, and excellent reconstruction performance.
- Summary
- Abstract
- Description
- Claims
- Application Information
AI Technical Summary
Problems solved by technology
Method used
Image
Examples
Embodiment Construction
[0022] The present invention will be further described below in conjunction with accompanying drawing.
[0023] refer to figure 1 , the specific implementation process of the present invention is as follows:
[0024] Step 1, divide an image into non-overlapping sub-image blocks, the sub-image block size of this example is ;
[0025] Step 2, for each sub-image block with The sampling rate is compressed and sampled, and the measured :
[0026] ,in is the first sub-image block pixel value, yes Gaussian random matrix, , is the number of compressed measurements;
[0027] Step 3, generate a value between 0 and 180 degrees Black and white edge images in one direction, calculate all the edge images The covariance matrix of the sub-image patches, generating Gaussian distribution with zero mean direction , using the DCT transform to generate the first direction Gaussian distribution ;
[0028] Step 4, in the first layer of the mixed model, by measuri...
PUM
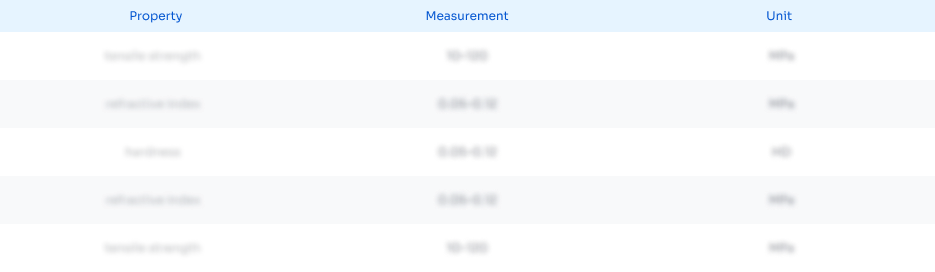
Abstract
Description
Claims
Application Information
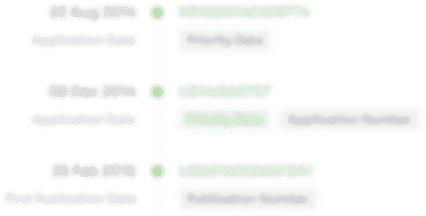
- R&D
- Intellectual Property
- Life Sciences
- Materials
- Tech Scout
- Unparalleled Data Quality
- Higher Quality Content
- 60% Fewer Hallucinations
Browse by: Latest US Patents, China's latest patents, Technical Efficacy Thesaurus, Application Domain, Technology Topic, Popular Technical Reports.
© 2025 PatSnap. All rights reserved.Legal|Privacy policy|Modern Slavery Act Transparency Statement|Sitemap|About US| Contact US: help@patsnap.com