Polarized SAR image classification method based deep multi-example learning
A multi-instance learning and classification method technology, applied in the field of polarimetric SAR image classification based on deep multi-instance learning, can solve the problem of low image classification accuracy, and achieve the effects of improving classification accuracy, improving feature information, and enriching feature information.
- Summary
- Abstract
- Description
- Claims
- Application Information
AI Technical Summary
Problems solved by technology
Method used
Image
Examples
Embodiment Construction
[0028] refer to figure 1 , the specific implementation steps of the present invention are as follows:
[0029] Step 1, input the polarimetric SAR image to be classified, and perform refined polarimetric Lee filtering on the image to obtain the polarimetric SAR image including the polarization coherence matrix T and the polarization covariance matrix C, which is realized by the following steps:
[0030] Step 1a, set the filter window size of the polarimetric SAR image to obtain multiple mean windows, select edge templates in different directions for each mean window, and perform edge detection to obtain multiple directional filter windows: in the polarimetric SAR classification image to be classified On the span total power image, the value of each pixel of the image is equal to the sum of the diagonal elements of the polarization covariance matrix of the pixel, and the size of the filter window is set to 7×7. According to the spatial position of the image pixel, from left to ...
PUM
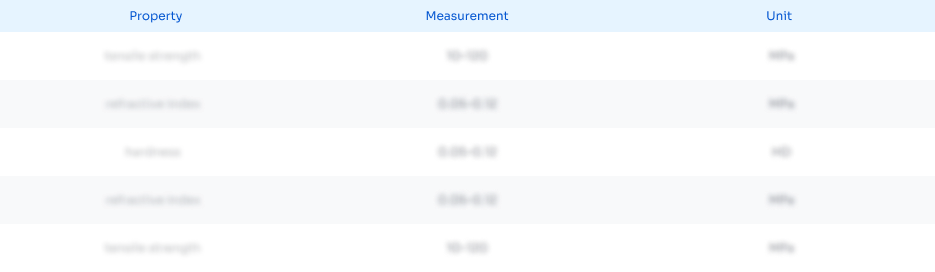
Abstract
Description
Claims
Application Information
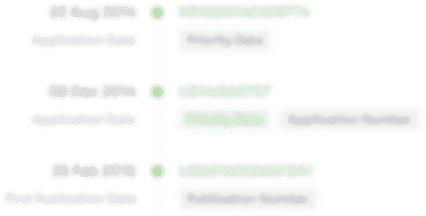
- R&D
- Intellectual Property
- Life Sciences
- Materials
- Tech Scout
- Unparalleled Data Quality
- Higher Quality Content
- 60% Fewer Hallucinations
Browse by: Latest US Patents, China's latest patents, Technical Efficacy Thesaurus, Application Domain, Technology Topic, Popular Technical Reports.
© 2025 PatSnap. All rights reserved.Legal|Privacy policy|Modern Slavery Act Transparency Statement|Sitemap|About US| Contact US: help@patsnap.com