Artificial neural network hardware implementation device based on probability calculation
An artificial neural network and probability calculation technology, applied in biological neural network models, physical realization, etc., can solve problems such as occupying connection resources, increasing power consumption, and large-scale hardware circuits of neural networks
- Summary
- Abstract
- Description
- Claims
- Application Information
AI Technical Summary
Problems solved by technology
Method used
Image
Examples
Embodiment 1
[0041] refer to figure 1 , a kind of artificial neural network hardware implementation device based on probability calculation in a preferred embodiment of the present invention, comprises input module, intermediate module and output module, and described input module comprises 1 input neuron (11), intermediate module It includes J interneurons (12), and the output module includes K output neurons (13), wherein, I, J, and K are all integers greater than or equal to 1. The input neuron (11) receives the first data (71) and outputs the first random data sequence (81). The interneuron (12) receives the first random data sequence (81) and the first random parameter sequence (51), and outputs the second random data sequence (82). The output neuron (13) receives the second random data sequence (82) and the second random parameter sequence (52), and outputs the second data (72). Among them, the first random data sequence (81), the second random data sequence (82), the first random ...
Embodiment 2
[0043] This embodiment is basically the same as Embodiment 1, and the special features are as follows:
[0044] Each interneuron (12) can use the first random data sequence (81) as an input variable and the first random parameter sequence (51) as a function parameter to complete the radial basis function operation, and the operation process uses the probability number (that is, the probability of 0 or 1 appearing in the data sequence within a period of time represents a numerical value), after the operation, the second random data sequence ( 82 ) will be output as the output data of the intermediate neuron ( 12 ). The types of the radial basis functions include, but are not limited to, Gaussian functions, multi-quadratic functions, inverse multi-quadratic functions, thin-plate spline functions, cubic functions, and linear functions.
Embodiment 3
[0046] This embodiment is basically the same as Embodiment 1, and the special features are as follows:
[0047] The first random data sequence (81), the second random data sequence (82), the first random parameter sequence (51), and the second random parameter sequence (52) can all be pseudo-random number sequences or true random number sequences. Its data width can be single-bit data width or multi-bit data width. Usually, the data in these sequences is one bit, that is, each data only needs one wire, which can greatly reduce the interconnection wires inside the network. However, in order to improve the calculation speed, these sequences can also use multi-bit data width to complete parallel calculations and speed up calculations.
[0048] Both the first random parameter sequence (51) and the second random parameter sequence (52) can be a sequence formed by a scalar parameter, or a sequence formed by a set of vector parameters. A scalar parameter means that the sequence rep...
PUM
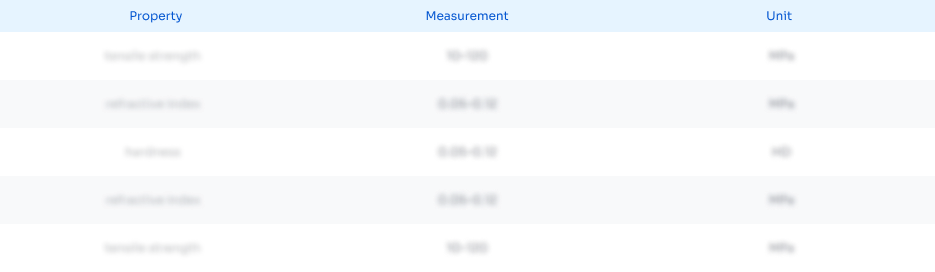
Abstract
Description
Claims
Application Information
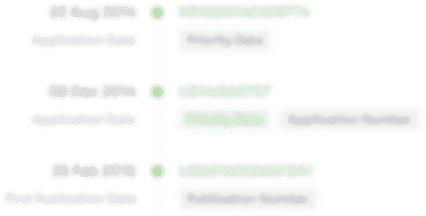
- Generate Ideas
- Intellectual Property
- Life Sciences
- Materials
- Tech Scout
- Unparalleled Data Quality
- Higher Quality Content
- 60% Fewer Hallucinations
Browse by: Latest US Patents, China's latest patents, Technical Efficacy Thesaurus, Application Domain, Technology Topic, Popular Technical Reports.
© 2025 PatSnap. All rights reserved.Legal|Privacy policy|Modern Slavery Act Transparency Statement|Sitemap|About US| Contact US: help@patsnap.com