A random neural network hardware realization device
A stochastic neural network and hardware implementation technology, applied in biological neural network models, physical realization, etc., can solve the problems of complex models and low accuracy of neural networks
- Summary
- Abstract
- Description
- Claims
- Application Information
AI Technical Summary
Problems solved by technology
Method used
Image
Examples
Embodiment 1
[0038] refer to figure 1 , image 3 , Figure 5 and Figure 7 , a schematic diagram of the system design of a random neural network hardware implementation device in a preferred embodiment of the present invention, which includes a three-layer structure—an input layer, a hidden layer and an output layer: the input layer consists of m input neurons I Each input neuron I includes a random number converter A, and the input vector (1) outputs random data sequence one (2) after passing through the random number converter A; the hidden layer consists of s hidden neurons J, Each hidden neuron J includes a random number converter B, a random function generator (41) and a deterministic number converter C. After passing through the random number converter B, the parameter code stream one (11) is combined with the random data sequence one. (2) After passing through the random function generator (41) together, the random data sequence two (13) is obtained, and the random data sequence ...
Embodiment 2
[0040] refer to figure 2 It is a schematic structural diagram of a hardware implementation device of a random neural network in a preferred embodiment of the present invention. The neural network includes m input neurons I, s hidden neurons J, and n output neurons K. The number of neurons in each layer is set according to different applications. There is an optimal number of input layer nodes m and The number of hidden layer nodes is s, which makes the network structure have high calculation accuracy. Input neuron I accepts input vector (1), passes through random number converter A, and outputs random data sequence one (2); implicit neuron J accepts random data sequence one (2), parameter code stream one (11), parameter Digital stream one (11) obtains random code stream sequence (12), random code stream sequence (12) and random data sequence one (2) through random number converter B and input it to random function generator (41) to obtain random data sequence Two (13), the ...
Embodiment 3
[0043] This embodiment is basically the same as the first embodiment, and the special features are as follows:
[0044] The hardware implementation device of a random neural network further includes a parameter code stream 1 (11) and a parameter code stream 2 (21), both of which are stored in a non-volatile memory. Among them, the parameter code stream 1 (11) is involved in calculating the output value of the hidden neuron J (13), the parameter code stream 2 (21) is involved in calculating the output value of the output neuron K (23), and the parameter code stream 1 (11) Can be random or non-random. When the parameter code stream one is a non-random sequence, the hardware implementation device for a random neural network further includes a random number converter B, which is used to convert the parameter code stream one (11) into a random sequence. The second parameter stream (21) can be a random sequence or a non-random sequence. When the second parameter code stream ( 21 )...
PUM
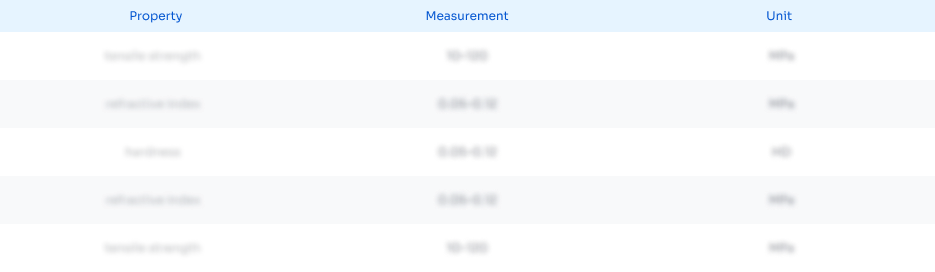
Abstract
Description
Claims
Application Information
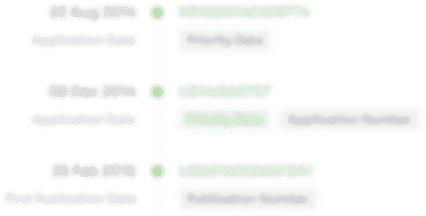
- R&D Engineer
- R&D Manager
- IP Professional
- Industry Leading Data Capabilities
- Powerful AI technology
- Patent DNA Extraction
Browse by: Latest US Patents, China's latest patents, Technical Efficacy Thesaurus, Application Domain, Technology Topic.
© 2024 PatSnap. All rights reserved.Legal|Privacy policy|Modern Slavery Act Transparency Statement|Sitemap