SAMME.RCW algorithm based face recognition optimization method
A face recognition and optimization method technology, applied in character and pattern recognition, computing, computer components, etc., can solve the problems of low recognition rate, improve quality, solve the problem of resampling, and improve the effect of classification accuracy
- Summary
- Abstract
- Description
- Claims
- Application Information
AI Technical Summary
Problems solved by technology
Method used
Image
Examples
Embodiment Construction
[0037] Provide the explanation of each detailed problem involved in the technical scheme of this invention below in detail:
[0038] The SAMME algorithm requires the correct rate of the weak classifier to be greater than 1 / k. The SAMME.R algorithm, on the basis of the SAMME algorithm, also requires that the weight of the correctly classified samples in each category be greater than the weight of any sample assigned to other classes. In order to ensure that in each weak classifier, the correctly classified samples account for the majority. From a vertical perspective, according to the theorem of large numbers, it ensures that after multiple iterations, the accuracy rate of the final integrated strong classifier is improved.
[0039] The SAMME.R algorithm restricts the weak classifiers obtained each time to ensure that the weights of correctly classified samples in each class are greater than the weights of any samples assigned to other classes. If this condition is met, continu...
PUM
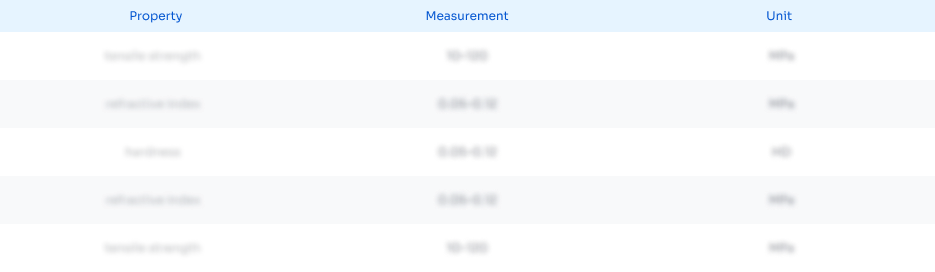
Abstract
Description
Claims
Application Information
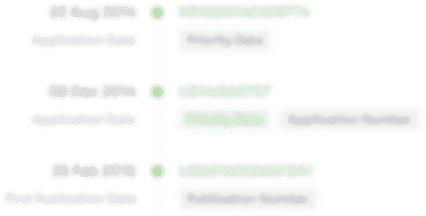
- Generate Ideas
- Intellectual Property
- Life Sciences
- Materials
- Tech Scout
- Unparalleled Data Quality
- Higher Quality Content
- 60% Fewer Hallucinations
Browse by: Latest US Patents, China's latest patents, Technical Efficacy Thesaurus, Application Domain, Technology Topic, Popular Technical Reports.
© 2025 PatSnap. All rights reserved.Legal|Privacy policy|Modern Slavery Act Transparency Statement|Sitemap|About US| Contact US: help@patsnap.com