Clustering method for attribute missing data set
A technology of missing data and clustering method, applied in the field of clustering of missing attribute data sets, can solve the problems of poor clustering accuracy of WDS and PDS, large estimation deviation, and low accuracy of clustering results.
- Summary
- Abstract
- Description
- Claims
- Application Information
AI Technical Summary
Problems solved by technology
Method used
Image
Examples
Embodiment 1
[0044] A clustering method for missing attribute data sets, clustering the missing data set S, the number of clustered categories is c, the missing data set S contains x data, the attribute dimension is y, and the number of missing attributes is n, the cluster center is expressed as a matrix with a size of c*y, and the clustering method includes the following steps:
[0045] S1. Perform ant colony coding on missing attributes and cluster centers: spatially superimpose all missing attributes in the data set and the attribute values of each dimension of each cluster center to form an n+c*y-dimensional vector, and put the The vector is used as the position vector of a single ant in the ant colony;
[0046] S2. Determine the missing attribute and the value space of the cluster center, the value space is the search range of the dimension corresponding to the position vector.
[0047] For the missing attribute, the nearest neighbor interval of the missing attribute is obtained by...
PUM
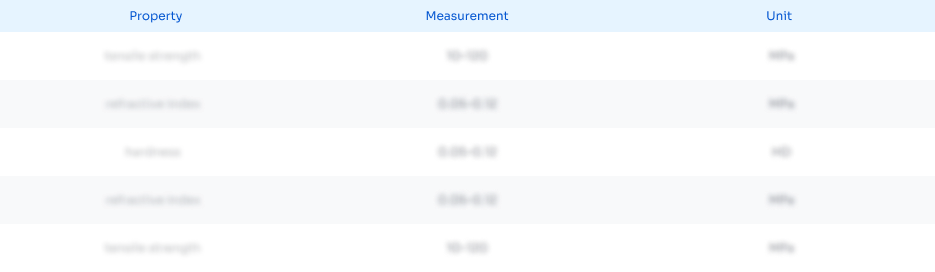
Abstract
Description
Claims
Application Information
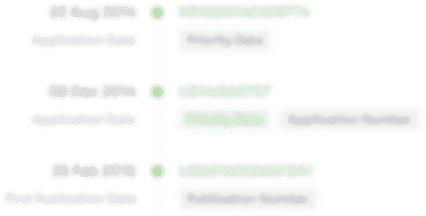
- R&D
- Intellectual Property
- Life Sciences
- Materials
- Tech Scout
- Unparalleled Data Quality
- Higher Quality Content
- 60% Fewer Hallucinations
Browse by: Latest US Patents, China's latest patents, Technical Efficacy Thesaurus, Application Domain, Technology Topic, Popular Technical Reports.
© 2025 PatSnap. All rights reserved.Legal|Privacy policy|Modern Slavery Act Transparency Statement|Sitemap|About US| Contact US: help@patsnap.com