Method and system for machine learning based assessment of fractional flow reserve
A deep neural network, object detection technology, applied in image data processing, instrumentation, computing, etc., can solve problems such as robustness of anatomical object detection
- Summary
- Abstract
- Description
- Claims
- Application Information
AI Technical Summary
Problems solved by technology
Method used
Image
Examples
Embodiment Construction
[0021] The present invention relates to methods and systems for anatomical object detection using edge-space deep neural networks. Embodiments of the invention are described herein to give an intuitive understanding of a machine learning based approach for anatomical object detection. Digital images usually consist of digital representations of one or more objects (or shapes). Digital representations of objects are generally described herein in terms of recognizing and manipulating objects. Such manipulations are intuitive manipulations implemented in the computer system's memory or other circuitry / hardware. Thus, it should be understood that embodiments of the present invention can be implemented within a computer system using data stored within the computer system.
[0022] Marginal Space Learning (MSL) is an efficient discriminative learning framework that can be used for anatomical object detection and tracking in medical images such as but not limited to computed tomogr...
PUM
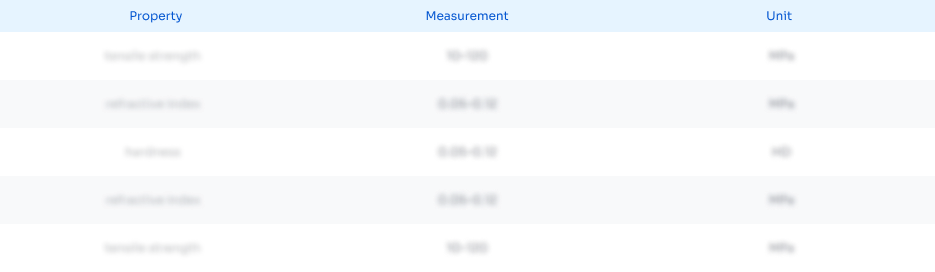
Abstract
Description
Claims
Application Information
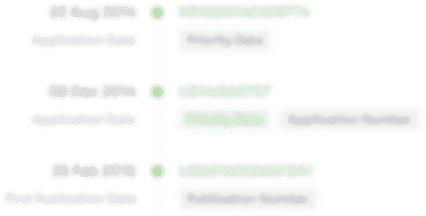
- R&D
- Intellectual Property
- Life Sciences
- Materials
- Tech Scout
- Unparalleled Data Quality
- Higher Quality Content
- 60% Fewer Hallucinations
Browse by: Latest US Patents, China's latest patents, Technical Efficacy Thesaurus, Application Domain, Technology Topic, Popular Technical Reports.
© 2025 PatSnap. All rights reserved.Legal|Privacy policy|Modern Slavery Act Transparency Statement|Sitemap|About US| Contact US: help@patsnap.com