Resting state function magnetic resonance image data classification method based on high-order super network
A technology of functional magnetic resonance and image data, applied in the field of image processing, can solve the problem of low classification accuracy, achieve the effect of high application value, solve the low classification accuracy and improve the classification accuracy
- Summary
- Abstract
- Description
- Claims
- Application Information
AI Technical Summary
Problems solved by technology
Method used
Image
Examples
Embodiment Construction
[0018] A method for classifying resting-state functional magnetic resonance imaging data based on a high-order hypernetwork, which is implemented by the following steps:
[0019] Step S1: Preprocess the resting-state fMRI images, and perform regional segmentation on the pre-processed resting-state fMRI images according to the selected standardized brain atlas, and then average time for each segmented brain region sequence extraction;
[0020] Step S2: Select a sliding window with a fixed length, and perform time window segmentation on the average time series of each brain region according to a certain step size;
[0021] Step S3: Calculate the Pearson correlation coefficient between the average time series of each brain region under each time window, thereby obtaining the Pearson correlation matrix;
[0022] Step S4: extracting the value of the corresponding element in the Pearson correlation matrix, thus obtaining the high-order correlation matrix;
[0023] Step S5: Using t...
PUM
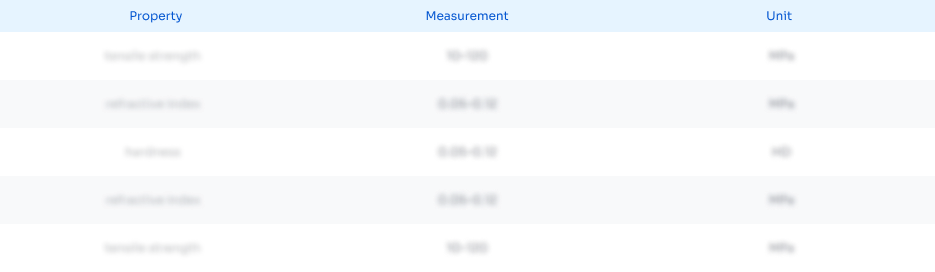
Abstract
Description
Claims
Application Information
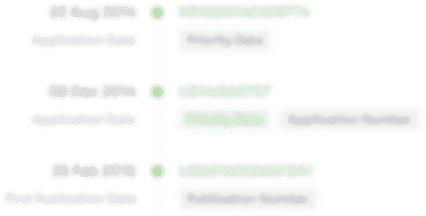
- Generate Ideas
- Intellectual Property
- Life Sciences
- Materials
- Tech Scout
- Unparalleled Data Quality
- Higher Quality Content
- 60% Fewer Hallucinations
Browse by: Latest US Patents, China's latest patents, Technical Efficacy Thesaurus, Application Domain, Technology Topic, Popular Technical Reports.
© 2025 PatSnap. All rights reserved.Legal|Privacy policy|Modern Slavery Act Transparency Statement|Sitemap|About US| Contact US: help@patsnap.com