Deep learning-based high-precision image fuzzy detection method
A technology of blur detection and deep learning, used in image enhancement, image analysis, image data processing, etc.
- Summary
- Abstract
- Description
- Claims
- Application Information
AI Technical Summary
Problems solved by technology
Method used
Image
Examples
Embodiment Construction
[0033] Below in conjunction with accompanying drawing, the present invention is described in detail:
[0034] The invention proposes a high-precision image blur detection method based on deep learning. Different from the existing method of extracting low-order features to optimize energy functions, the present invention extracts high-order features of images extracted by CNN from multiple scales for classification, and can realize high-precision image blur detection. This technology combines deep convolutional neural networks (ConvolutionalNeural Networks
[0035] Such as figure 1 Shown, the present invention adopts following technical scheme:
[0036] Step 1 (110, 120, 130) establishes a deep convolutional neural network model CNN and initialization, and inputs a detection image to it;
[0037] Among them, the overall architecture of the deep convolutional neural network is as follows: figure 2 As shown, the overall framework of blur detection at multiple scales is demo...
PUM
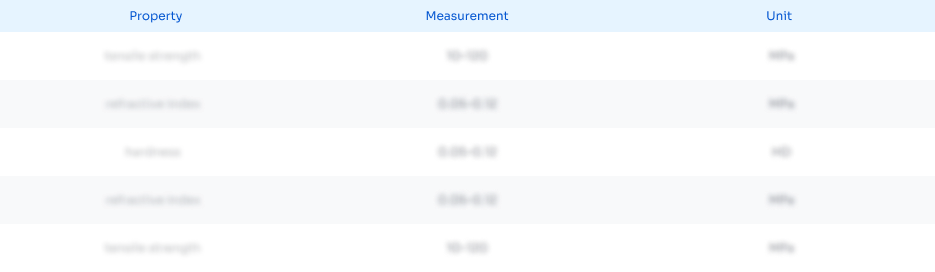
Abstract
Description
Claims
Application Information
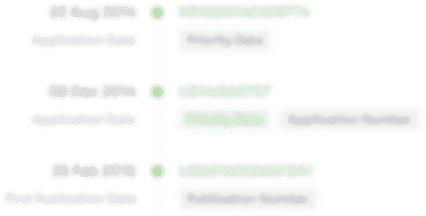
- R&D Engineer
- R&D Manager
- IP Professional
- Industry Leading Data Capabilities
- Powerful AI technology
- Patent DNA Extraction
Browse by: Latest US Patents, China's latest patents, Technical Efficacy Thesaurus, Application Domain, Technology Topic, Popular Technical Reports.
© 2024 PatSnap. All rights reserved.Legal|Privacy policy|Modern Slavery Act Transparency Statement|Sitemap|About US| Contact US: help@patsnap.com