Active learning based data automatic marking method
An automatic labeling and active learning technology, applied in the field of active learning, can solve the problems of complexity, large manual labor, and high marking cost, and achieve the effect of shortening time and overhead.
- Summary
- Abstract
- Description
- Claims
- Application Information
AI Technical Summary
Problems solved by technology
Method used
Image
Examples
Embodiment 1
[0048] refer to figure 1 , figure 1 A text data labeling system based on active learning provided for this embodiment, specifically includes:
[0049] 101 Processing the marked text data set flag and the unmarked text data set imflag: clustering the marked text data flag, and marking the value of the center point of each cluster;
[0050] The clustering processing of the marked text data set flag refers to clustering the marked text data set flag samples according to the label value of each text data sample, and dividing the samples that are similar and of the same category into one category. The number of clusters in the experimental clustering is k, and the set of clusters is expressed as {f 1 , f 2 ,..., f k}, and calculate the value {a of the cluster center point of each cluster 1 ,a 2 ,...,a k}.
[0051] The unlabeled data set imflag uses a cluster-based linear scan search, and the special search method is aimed at reducing the number of calculations for the very ...
PUM
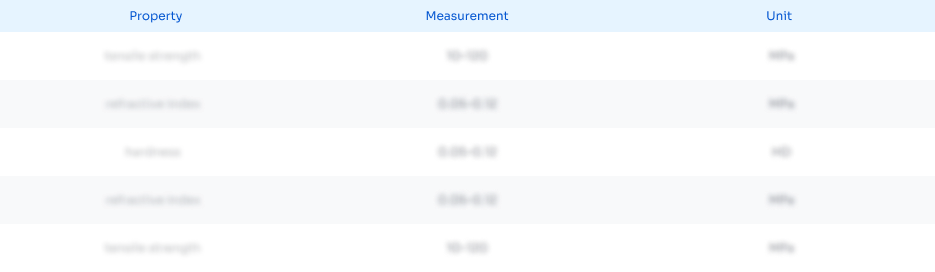
Abstract
Description
Claims
Application Information
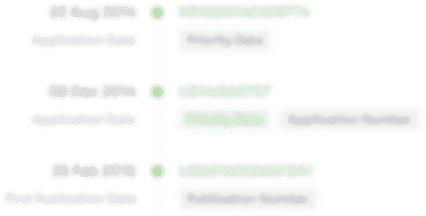
- R&D Engineer
- R&D Manager
- IP Professional
- Industry Leading Data Capabilities
- Powerful AI technology
- Patent DNA Extraction
Browse by: Latest US Patents, China's latest patents, Technical Efficacy Thesaurus, Application Domain, Technology Topic, Popular Technical Reports.
© 2024 PatSnap. All rights reserved.Legal|Privacy policy|Modern Slavery Act Transparency Statement|Sitemap|About US| Contact US: help@patsnap.com