Method for forecasting traffic flow in urban area based on depth learning
A traffic flow and urban area technology, applied in the field of urban area traffic flow prediction based on deep learning, can solve the problem of not considering the temporal and spatial characteristics of traffic flow changes at the same time, lacking support for long-term traffic flow forecasting methods, and only considering the correlation of traffic flow changes, etc. problem, to achieve the effect of improving the prediction accuracy
- Summary
- Abstract
- Description
- Claims
- Application Information
AI Technical Summary
Benefits of technology
Problems solved by technology
Method used
Image
Examples
Embodiment
[0057] Such as figure 1 Shown is the algorithm flow chart of the traffic flow prediction method in urban areas based on deep learning, and its prediction system includes a traffic flow calculation module and a traffic flow prediction module based on grid areas. In the traffic flow calculation module, firstly, the urban road network is divided into grid areas according to latitude and longitude, and the license plate recognition (LPR) equipment of each road section in the urban road network is mapped to the grid area; secondly, according to the data of the LPR equipment, the The vehicle passing records of each device in each time period; again, the results of the above two steps are combined to calculate the traffic flow in each grid area in each time period; finally, the traffic data is normalized. In the traffic flow prediction module, firstly, the traffic flow data in the key time period is selected as the input of the convolutional long short-term memory network (ConvLSTM),...
PUM
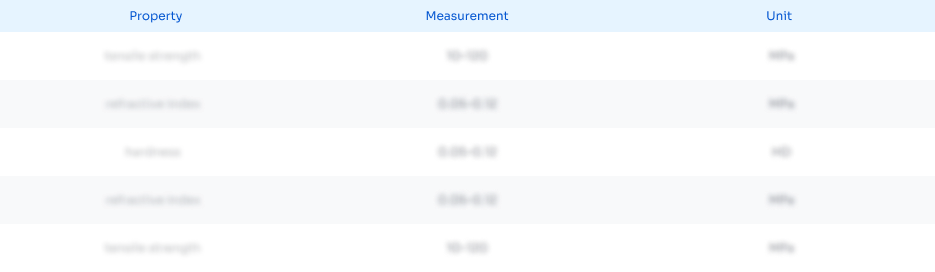
Abstract
Description
Claims
Application Information
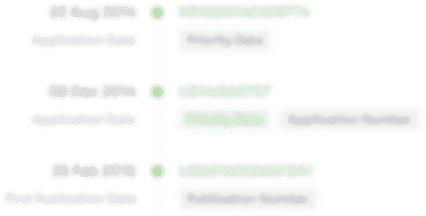
- R&D Engineer
- R&D Manager
- IP Professional
- Industry Leading Data Capabilities
- Powerful AI technology
- Patent DNA Extraction
Browse by: Latest US Patents, China's latest patents, Technical Efficacy Thesaurus, Application Domain, Technology Topic, Popular Technical Reports.
© 2024 PatSnap. All rights reserved.Legal|Privacy policy|Modern Slavery Act Transparency Statement|Sitemap|About US| Contact US: help@patsnap.com