MGKFCM (multipath Gauss kernel fuzzy c-means clustering algorithm)
A mean value clustering and multi-path technology, applied in computing, computer components, character and pattern recognition, etc., can solve problems such as poor clustering effect, and achieve the effect of improving classification performance
- Summary
- Abstract
- Description
- Claims
- Application Information
AI Technical Summary
Problems solved by technology
Method used
Image
Examples
Embodiment Construction
[0038] In this embodiment, three types of two-dimensional Gaussian data sets are constructed for algorithm comparison test, and the centers of the three types of two-dimensional Gaussian data sets are respectively (5,5), (10,5), (7.59.5826), which The three centers form an equilateral triangle, that is, the centers of the three types of data sets are equidistant, and the covariance matrices of the three types of Gaussian data sets are all [20,02], and the covariance matrix is used to reflect the degree of dispersion of the data set , the number of samples in the three data sets is all taken as 50. Because the multi-path Gaussian kernel fuzzy c-means clustering algorithm (hereinafter referred to as the MGKFCM algorithm) combines the advantages of the GKFCM algorithm and the PSO-KFCM algorithm, in order to verify the effectiveness of the MGKFCM algorithm, the MGKFCM algorithm is combined with the GKFCM algorithm and the PSO-KFCM algorithm. Do a comparison test.
[0039] The m...
PUM
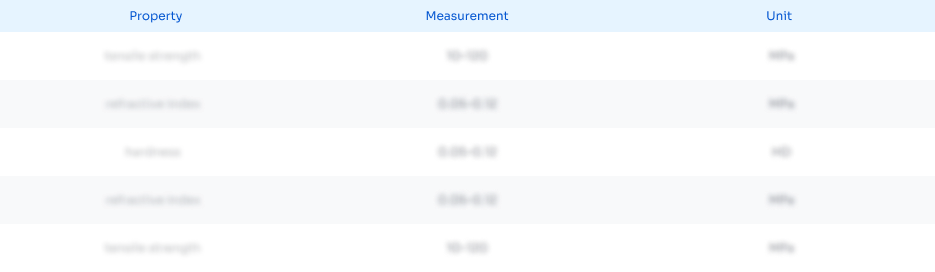
Abstract
Description
Claims
Application Information
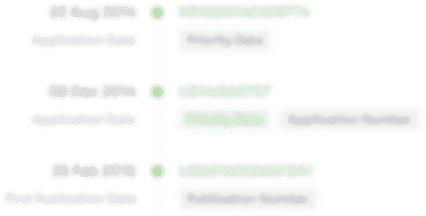
- R&D Engineer
- R&D Manager
- IP Professional
- Industry Leading Data Capabilities
- Powerful AI technology
- Patent DNA Extraction
Browse by: Latest US Patents, China's latest patents, Technical Efficacy Thesaurus, Application Domain, Technology Topic.
© 2024 PatSnap. All rights reserved.Legal|Privacy policy|Modern Slavery Act Transparency Statement|Sitemap