Self-paced-cooperative training learning method
A technology for collaborative training and learning methods, applied in the field of multi-view semi-supervised learning models, which can solve problems such as lack of model explanations
- Summary
- Abstract
- Description
- Claims
- Application Information
AI Technical Summary
Problems solved by technology
Method used
Image
Examples
Embodiment 1
[0078] Table 1 is an explanatory table for six groups of text data.
[0079] Table 1: Experimental data of Example 1
[0080]
[0081]
[0082] The six text data sets shown in Table 1 are used as the experimental objects of the present invention, and all samples are manually divided into two fields of view. Each dataset has two categories whose structural characteristics are illustrated in Table 1.
[0083] Table 2 is the accuracy table for classification using seven semi-supervised methods including the present invention on six groups of text data.
[0084]
[0085] see figure 1 , step S1: read the text data, for the first data set in Table 1, select 2 respectively from positive samples and negative samples k , 3.2 k samples are labeled samples, and the remaining samples are unlabeled samples. For the second, third, and fourth data sets in Table 1, select 2 for the positive and negative classes respectively k , 6.2 k samples are labeled samples. For the last ...
Embodiment 2
[0135] Table 3 is the accuracy table of Person re-Identification using three multi-view semi-supervised methods including the invention on the Market-1501 dataset.
[0136]
[0137] In this example, the Market-1501 dataset is used for the Person re-identification task. Personre-ID refers to a class of tasks where, for a person captured by a camera, it is necessary to determine whether the person is captured by other cameras. The Market-1501 dataset includes 32668 photos of 1501 individuals. Each person's photo is captured by a maximum of six cameras and a minimum of two cameras. Here, 12,936 clipped photos containing 751 people are selected as the training data set, and 19,732 clipped photos containing 750 people are selected as the test data set.
[0138] Next, feature extraction is performed on the training data and test data sets. In order to obtain different features, different networks such as caffenet, Googlenet, and Vggnet are used here. The features extracted by ...
PUM
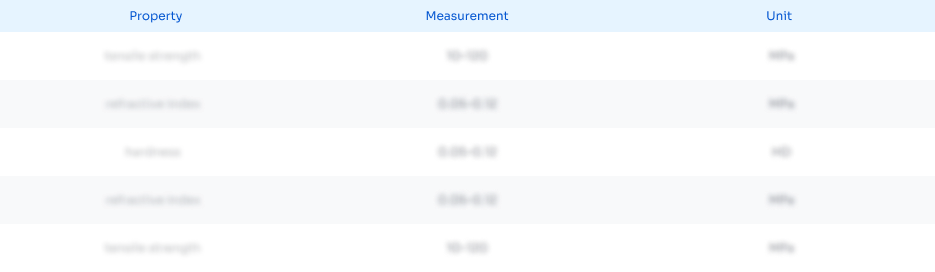
Abstract
Description
Claims
Application Information
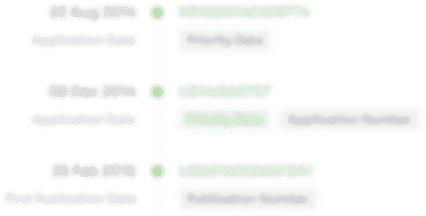
- R&D Engineer
- R&D Manager
- IP Professional
- Industry Leading Data Capabilities
- Powerful AI technology
- Patent DNA Extraction
Browse by: Latest US Patents, China's latest patents, Technical Efficacy Thesaurus, Application Domain, Technology Topic, Popular Technical Reports.
© 2024 PatSnap. All rights reserved.Legal|Privacy policy|Modern Slavery Act Transparency Statement|Sitemap|About US| Contact US: help@patsnap.com