RGB-D object identification method
An object recognition and object technology, applied in the fields of stereo vision and deep learning, can solve the problems of imperfect feature description and low recognition accuracy, and achieve the effect of solving the poor recognition effect.
- Summary
- Abstract
- Description
- Claims
- Application Information
AI Technical Summary
Problems solved by technology
Method used
Image
Examples
Embodiment 1
[0024] The embodiment of the present invention combines multiple data modes such as color images, depth images, grayscale images, and surface normal vectors, and proposes an RGB-D object recognition method. The specific operation steps are as follows:
[0025] 101: Obtain a grayscale image generated from a color image and a surface normal vector generated from a depth image, and use the color image, grayscale image, depth image, and surface normal vector together as multi-data mode information;
[0026] 102: Extract high-level features in color images, grayscale images, and surface normal vectors through convolution-recurrent neural networks;
[0027] 103: Using convolution-Fisher vector-recurrent neural network to extract high-level features of depth images;
[0028] 104: Perform feature fusion of the above-mentioned multiple high-level features to obtain the total features of the object, and input the total features of the object into the feature classifier to realize the ob...
Embodiment 2
[0031] The scheme in embodiment 1 is further introduced below in conjunction with specific calculation formulas and examples, see the following description for details:
[0032] 201: Obtain multi-data mode information;
[0033] In order to more fully mine the color image and depth image information, the embodiment of the present invention adds two data modes, that is, the grayscale image generated by the color image and the surface normal vector generated by the depth image, so as to provide more information for object recognition. useful information. Specifically, the depth image and surface normal vector can provide the geometric information of the object, and the color image and grayscale image can provide the texture information of the object.
[0034] 202: Use convolution-recurrent neural network to extract high-level features of color images, grayscale images, and surface normal vectors;
[0035] Among them, the Convolutional-Recursive Neural Network (CNN-RNN) model co...
Embodiment 3
[0061] Combine below figure 2 The scheme in embodiment 1 and 2 is carried out feasibility verification, see the following description for details:
[0062] figure 2 The visualization result of this method is given, that is, the confusion matrix of the recognition result. Among them, the horizontal axis of the confusion matrix represents the predicted object category, a total of 51 categories, such as apple, bowl, cereal__box, etc., and the vertical axis represents the real object category in the data set.
[0063] The value of the diagonal element of the confusion matrix represents the recognition accuracy of this method in each category, such as the value of the element in row a and column b represents the percentage of misidentifying objects of type a as objects of type b. from figure 2 It can be seen that this method has obtained better recognition results, and most categories can obtain higher recognition rates.
PUM
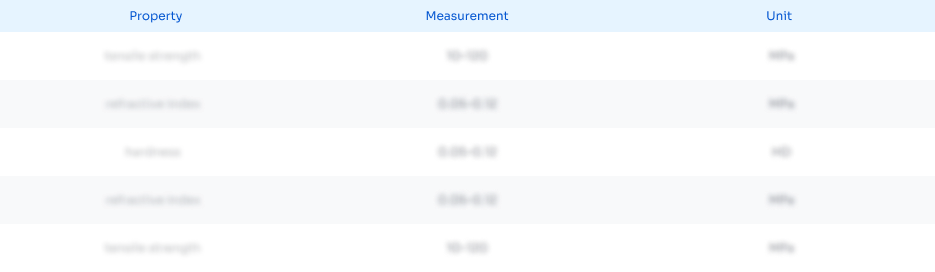
Abstract
Description
Claims
Application Information
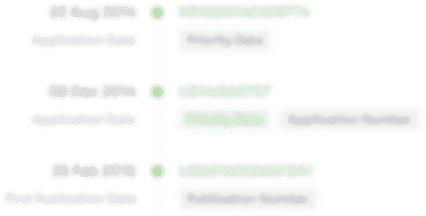
- R&D Engineer
- R&D Manager
- IP Professional
- Industry Leading Data Capabilities
- Powerful AI technology
- Patent DNA Extraction
Browse by: Latest US Patents, China's latest patents, Technical Efficacy Thesaurus, Application Domain, Technology Topic.
© 2024 PatSnap. All rights reserved.Legal|Privacy policy|Modern Slavery Act Transparency Statement|Sitemap